This article delves into the EU’s groundbreaking rules for general-purpose AI (GPAI) models, as outlined in the politically agreed-upon AI Act on December 8th. It scrutinizes key questions, including whether this approach deviates from the original risk-based proposal, navigates the complexities of risk management in foundational models, and grapples with the uncertainties in benchmarking methods. Additionally, it explores the roles of codes of conduct and the Digital Services Act (DSA) in this context and delves into the open-source landscape within AI model regulation. While this agreement maintains flexibility in the ever-evolving AI landscape, it faces challenges such as aligning systemic risks with highly capable models, addressing inconsistencies in model categorization, and the potential overlap of regulatory tools. It also underscores the need for more clarity regarding systemicity of risks and benchmarks, and more nuances in the realm of open models. Supervising advanced models demands substantial resources and effort, making this a challenging task. As stakeholders in the Brussels bubble know: The deal is not done until it’s done: this article is released as a beta version and will be adapted once the text is available, and recitals are final.
In 2021, the Commission published its proposal for the AI Act,[1] accompanied by an impact assessment.[2] The European Commission’s proposal sought to regulate the development, deployment, and use of artificial intelligence in the EU to ensure safety and fundamental rights. It categorizes different levels of risk and prescribes regulatory measures accordingly, i.e., the prohibition of certain use cases associated with ‘unacceptable’ risks, stringent rules for high-risk uses, and transparency rules for certain low risk uses. In the assessment, it estimates that high-risk applications would comprise no more than 5% to 15% of all AI applications, to counter criticism of overregulating a nascent market.
The emergence of foundation models[3] has altered the trajectory of AI, AI applications and related risks. These models, which have evolved from deep learning breakthroughs over the last decade,[4] are now the source of an unprecedented AI hype. 2023 has been the year of phenomenal AI advancement and exponential growth in their capabilities, market reactions from venture capitalists and cloud providers that have enabled AI labs to acquire costly computing resources through their investments, and worldwide regulatory activities.[5] These trends were accompanied by prominent litigation cases over IP and data protection infringements[6] as well as defamation claims,[7] adaptation of user policies in a quest for more training data[8] and copyright indemnity commitments by the largest and best funded model providers or AI platform/cloud providers for their commercial users.[9] Last, we have seen an increase in AI incidents,[10] including the discovery of hundreds of instances of exploitative mages of children in a public dataset used for AI text-to-image generation models.[11]
Although their operational mechanism continues to rely on predicting successive words from vast internet data, these models now compete with human performance on many academic and professional benchmarks.[12] Concerns about the risks of AI models have been prominently voiced by respected researchers,[13] including those who have significantly contributed to the breakthroughs in the deep learning paradigm. Additionally, research indicating that continued scaling of these models could unpredictably enhance their capabilities[14] has intensified the urgency for AI regulation, not just in Europe but globally.[15]
Foundation models have also increased the complexity of the AI value chain by introducing new types of services that facilitate interactions directly between model developers and end-users through application programming interface .[16] It is, thus, not surprising that the advent of generative AI has unsettled the legislative progression of the proposed AI Act, compelling lawmakers to re-evaluate the categorization and allocation of responsibilities between model developers, AI system providers and deployers of AI systems (called users in the original proposal). Whether or not to regulate upstream foundation model providers was and remains one of the most contentious and heavily lobbied topics in trilogue discussions under the Spanish presidency before a political agreement was reached on 8 December.
I. The provisional agreement on GPAI/foundation models[17]
The provisional agreement introduces a new risk category, systemic risks; it defines obligations for GPAI[18] models and was guided by the European Commission[19] as well as proposals put forward by the Spanish presidency.[20] It leaned on substantive elements of the Parliament’s resolution, the structural approach of the Council and elements of the Digital Services Act (DSA)[21] and Digital Market Acts (DMA).[22] It entails new obligations for all GPAI models and additional obligations for GPAI models entailing systemic risks, thereby adding a new risk category to the existing risk categories of the AIA (Prohibited Artificial Intelligence Practices (Title II), High-Risk AI Systems (Title III), Transparency Obligations for Certain AI Systems (Title IV)).
GPAI models are now regulated in a tiered approach with more obligations for those GPAI model providers that entail systemic risks. All providers of GPAI – independent of the risk they pose – must create and regularly update the technical documentation of their model. This includes details of its training and testing processes, along with the results of its evaluation. Providers of GPAI must also prepare and update documentation for AI system providers who plan to integrate the GPAI model into their systems to help these providers understand the AI model’s capabilities and limitations and comply with the regulation. Providers are required to establish a policy for complying with EU copyright law and draft and publicly share a detailed summary of the content used for training the AI model. They must also cooperate with the Commission and national authorities in executing their duties under the regulation.
GPAI providers with systemic risks must in addition perform model evaluation, assess, and mitigate possible systemic risks, monitor, and report serious incidents, adopt corrective measures, and ensure an adequate level of cybersecurity protection for the model and its physical infrastructure.
A GPAI model falls in the category entailing systemic risks if it has high impact capabilities evaluated on benchmarks or per decision by the AI Office. The risk must be specific to the high impact capabilities. They are presumed to have high impact when the cumulative amount of compute used for its training exceeds 10^25 floating point operations (FLOPs),[23] or to be defined benchmarks indicate so. Developers can challenge a designation if a model, due to its specific characteristics, does not present systemic risks.
The Commission has the authority to adopt delegated acts to amend the thresholds and to supplement benchmarks and indicators in response to evolving technological developments, such as advancements in algorithms or improved hardware efficiency.[24] The Commission may also consider the number of tokens and parameters used in the model,[25] the modality of the model, its reach (presumed to have high impact if made available to at least 10 000 registered business users established in the EU), and the number of registered end-users.[26]
All obligations are further specified in detailed annexes.[27] Providers can demonstrate compliance through codes of practice which will be facilitated by the AI Office until a harmonized standard is published. The adherence to a European harmonized standard gives providers a presumption of conformity. Providers of AI models with systemic risks not adhering to an approved code of practice must show alternative means of compliance for Commission approval. An AI Office within the Commission is tasked to oversee these most advanced AI models, contribute to fostering standards and testing practices, and enforce the common rules in all member states. A scientific panel of independent experts will advise the AI Office about GPAI models, by contributing to the development of methodologies for evaluating the capabilities of foundation models, advising on the designation and the emergence of high impact foundation models, and monitoring possible material safety risks related to foundation models.
The AI Board, which would comprise member states’ representatives, will remain as a coordination platform and an advisory body to the Commission and will give an important role to Member States on the implementation of the regulation, including the design of codes of practice for foundation models. Finally, an advisory forum for stakeholders, such as industry representatives, SMEs, start-ups, civil society, and academia, will be set up to provide technical expertise to the AI Board.
The validity of the claim that the introduction of rules for foundation models represents a departure from the risk-based approach remains elusive. Risk regulation aims to strike a fair balance between the various economic and constitutional interests purported by the Union in the regulation of the Digital Single Market. [28]
The much-debated structure of the AIA follows the ‘top-down’[29] approach of European harmonized product safety regulation and concentrates its regulatory attention on the AI system provider, akin to manufacturer in safety legislation. Previous discussions during the legislative process highlighted the need to redirect oversight towards users/deployers of AI systems. This shift was considered crucial because decisions made during the deployment of AI systems can significantly influence the system’s performance and, in turn, impact the rights of individuals potentially affected by these systems. In the original proposal, model providers, though fundamental components of an AI system, were only required to collaborate and were not specifically targeted beyond this duty: “In the light of the complexity of the artificial intelligence value chain, relevant third parties, notably the ones involved in the sale and the supply of software, software tools and components, pre-trained models and data, or providers of network services, should cooperate, as appropriate, with providers and users to enable their compliance with the obligations under this Regulation and with competent authorities established under this Regulation.”[30] The Commission considered that AI system providers that develop a system for a high-risk use case would procure information necessary to comply with their legal obligations, at the time with mostly narrow AI models trained for specific tasks in mind (e.g., classification of images and text).[31]
The shift in paradigm towards foundation models, coupled with growing awareness of upstream risks when using them in infinite new ways, initiated the debate on appropriate model regulation. While the Slovenian presidency explicitly excluded GPAI,[32] the French presidency took a different approach by introducing a new title for GPAI systems. This addition extended certain high-risk use case obligations to AI system providers, if used in high-risk scenarios.[33] In December 2022, under the Czech presidency, the Council adopted its negotiation position for trialogue.[34] The compromise followed the previous extension of certain high-risk AI requirements to GPAI providers but left details to an implementing act, thereby avoiding a clash between increasingly diverging views on this matter amongst Member States. Overall, the approach of the Council continued to be limited to high-risk uses. Model providers would be able to avoid regulation by excluding high-risk uses in their use policies. The evolving debate reflects not only the significant advancements in the field but also indicates that legislators have improved their knowledge and understanding of these technologies.
The general approach was agreed on 6 December 2022, just days after the launch of ChatGPT which by January 2023 gained over 100 million users and was then the fastest-growing consumer software application in history.[35]
The slower progress of the file in the European Parliament provided an opportunity for it to thoroughly consider new AI advancements and keep pace with rapidly evolving discussions in international AI governance. In its negotiating position adopted at the Strasbourg’s plenary session of June 14th, 2023,[36] all AI system providers and foundation models were under an obligation to follow key principles such as ensuring human agency and oversight, maintaining technical robustness and safety, adhering to privacy and data governance, ensuring transparency, promoting diversity, non-discrimination, and fairness, and focusing on social and environmental well-being. In addition, under the title concerning high-risk uses, foundation models were required to be designed, tested, and analyzed in a way that identifies, reduces, and mitigates foreseeable risks to health, safety, fundamental rights, the environment, democracy, and the rule of law, with involvement from independent experts and documentation of any non-mitigable risks post-development. Generative foundation models would have to comply with additional transparency requirements, including the disclosure that content generated by AI, designing the model to prevent it from generating illegal content and publishing summaries of copyrighted data used for training. These rules sparked contention among political parties, and their integration into Title II (addressing high-risk uses) led to inconsistencies, but the shift in focus towards models reflected a deeper understanding of AI’s complexities and the underlying paradigm change, and evolving priorities in AI governance.
The inclusion of specific obligations for managing systemic risks in highly capable models marks an expansion of risk categories. By their very nature, systemic risks are not restricted to just one particular use case or application. This broadens the scope of regulatory attention, but it does not depart from the risk-based approach. Rather, this reflects the pace of AI technology advancements and the concerted efforts of legislators to stay abreast with the developments, specifically within the context of the AI Act.
In addition, risk mitigation mechanisms employed in AI foundation models bear a resemblance to content moderation policies in companies, in that both aim at identifying and addressing potential issues proactively. Just as content moderation policies in companies filter and manage online content to maintain standards and safety, risk mitigation in AI models involves implementing guardrails to ensure the models operate within ethical and operational guidelines. The structure of the AIA GPAI provisions now follows the approach taken in the DSA for online platforms, with general due diligence obligations for all platforms, respectively general transparency obligations for all foundation models, and heightened obligation for very large online platforms (VLOPs) and very large search engines (VLOSEs), respectively very capable models. In contrast to the DSA, where challenging the designation has to go through court,[37] the provisional agreement introduces a rebuttal process similar to that of the DMA’s rebuttal regarding gatekeeper designations.[38] It allows the provider of a GPAI model to challenge the designation if it sufficiently substantiates that the specific GPAI model does not actually present systemic risks typically associated with models that meet the standard criteria. The rationale behind including a rebuttal right might stem from the limited knowledge currently available about the systemic risks uniquely associated with such highly capable models.
General transparency obligations, now uniquely imposed for GPAI models, are not in contrast to the original proposal, which emphasized collaboration duties. Conversely, this prompts the question of whether deployers integrating narrow AI models could also benefit from similar transparency rules, given the continued significance of narrow AI models within the AI ecosystem. These models, defined by their specialized and restricted functions, offer a stark contrast to the broader scope of GPAI I systems. Despite this, they still encounter similar known risks, such as the presence of biased data sets. This observation highlights a potentially missed opportunity to enhance market transparency in this area. Furthermore, the reasoning for differentiating general information obligations between AI system providers integrating either GPAI or narrow AI models remains somewhat obscure. Providers of high-risk AI systems using narrow AI models remain obligated to establish contractual agreements, with the aim of addressing any informational or collaborative gaps.
Overall, there is no substantial deviation from the established risk-based approach. However, this does not imply that the provisions are without burdens or excessively bureaucratic. Yet, it’s important to recognize that this provision targets a small number of players but benefits the entire ecosystem that integrates models into their systems. In addition, this will significantly facilitate insights for individuals affected by AI-assisted decision-making when seeking redress. Clarity will also need to be added regarding liability rules, encompassing both the product liability and the AI liability directives. [39]
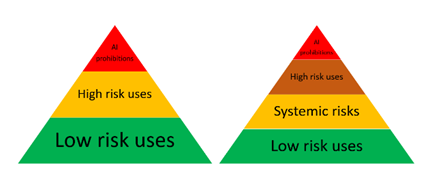
III. Which threshold for what systemic risk?
It is commonly understood that increasing capabilities also increases risks. It is less clear how to measure it.
III.1 What are highly capable models?
The high capability presumption. ‘High-impact capabilities’ in GPAI models is defined as capabilities that match or exceed the capabilities recorded in the most advanced GPAI I models. Roughly, it attempts to capture current ‘frontier’ models, [40] those that the newly established Frontier Model Forum is concerned with.[41] A rough method for calculating the FLOP/s used by an application involves estimating the FLOPS capacity of the computers used and multiplying this by the duration in seconds for which each computer was active during training.[42] A discussion to use FLOP/s for describing a threshold of systemic AI risks in foundation models entered the debate of the legislative process late. In trilogue, the EU tiered approach’s exact thresholds were debated between 10^21 to 10^26 FLOP,[43] and ultimately set at 10^25. The suggestion to regulate capable models via compute measurements was introduced by the Center for AI Governance.[44] The introduction of an exact threshold to regulate emerging (dangerous) capabilities has also been suggested in a prominent paper in July 2023.[45] Therein it is stated that “one simple approach would be to say that any foundation model trained with more than some amount of computational power – for example 10^26 FLOP – has the potential to show sufficiently dangerous capabilities.” This ‘simple’ approach has been adopted in the present regulation, yet it is not clear if ‘sufficiently dangerous capabilities’ directly correspond to or are different from ‘high impact capabilities’ and systemic risks, and how the lower threshold relates.
A key uncertainty arises in calculating FLOP/s, as models with fewer parameters may imply lower computational complexity, and a reduction in FLOP/s. In addition, research has shown that current large language models (LLMs) are significantly undertrained, a consequence of their focus on scaling language models whilst keeping the amount of training data constant.[46] Smaller models, when trained with more extensive and higher-quality datasets, can already today outperform larger models with more parameters,[47] confirming the expected trend in the coming year towards smaller, yet more powerful AI models that require less computing power. A FLOP threshold methodology will thus decay over time.[48]
The compromise text assumes systemic risks for models exceeding 10^25 FLOPs but allows for periodic adaptation and grants the Commission’s AI Office with advice from a scientific panel of independent experts leeway to identify providers using alternative criteria to classify a GPAI model as systemically risky. These criteria include benchmarks, assessments of the models’ capabilities, and the count of registered end-users. Given the uncertainty on FLOP calculation, the Commission may revert to other criteria to define high-impact capabilities.
Other defining factors for high capability. Multiple benchmarks and leaderboards have been rapidly emerging, most prominently MT Bench Leaderboard, AlpacaEval Leaderboard, the Chatbot Arena (LMSYS Org), and the Open LLM Leaderboard (Hugging Face).[49]
An example of an evaluation of capabilities (and potential risks) of foundation models, focused on language, is the HELM project.[50] HELM (Holistic Evaluation of Language Models) is a framework designed to improve the transparency and understanding of language models in cooperation with the most advanced AI labs. It encompasses a wide range of scenarios and metrics for evaluating these models, focusing on seven key metrics: accuracy, calibration, robustness, fairness, bias, toxicity, and efficiency. HELM evaluates a broad subset of scenarios and adopts a multi-metric approach to ensure comprehensive assessment. It aims to provide a more complete characterization of language models, highlighting their capabilities, limitations, and trade-offs across different models and metrics.[51]
The HELM project indicates two important challenges in benchmarking: access to data and costs.[52] These benchmarks are accompanied by uncertainties and criticism, which are inherent to the contextual nature of language.[53] Furthermore, the prevalent reliance on benchmarks in AI research has given rise to competitive and collaborative dynamics that remain largely unexplored but may have an impact on state-of-the-art performance.[54] This aspect must be considered when opting for a formal designation by the Commission/AI Office. Within this context, it is important to highlight the significance of providing data access to qualified researchers for the assessment of closed high-impact AI models during benchmarking. However, it is worth noting that access regulations for advanced AI models, particularly for risk evaluation purposes, are noticeably lacking.[55]
III.2 From risks of highly capable models to systemic risks
The range of risks. A systemic risk at Union level means a risk that is specific to the high-impact capabilities of GPAI models, having a significant impact on the internal market due to its reach, and with actual or reasonably foreseeable negative effects on public health, safety, public security, fundamental rights, or the society as a whole, that can be propagated at scale across the value chain. Would many known risks in smaller models be excluded from consideration of systemicity, even when they become systemic, if only risks specific to advanced models are considered? OpenAI’s GPT4 system card release, for example, provides some insights into emerging risks specific to GPT4 which we would assume here to be captured by the threshold.[56] The card lists risks such as hallucinations, harmful content, harms of representation, allocation and quality of service (i.e., bias), disinformation and influence of operations, proliferation of conventional and unconventional weapons, privacy, cybersecurity, potential for risky emergent behaviors, interactions with other systems, economic impacts, acceleration, and overreliance. However, most risks have been identified in smaller predecessors. A risk that was newly observed in these advanced models is its interaction with other systems. By chaining these systems together, the model was able to find risky alternatives to what has been guard railed in the model itself.[57] Other risks remain hypothetical with increased model capabilities but have not yet been observed (self-replication).[58]
There is, thus, ambiguity in which risks are considered as risks specific to advanced models and how capabilities of models correlate with specific risks. Furthermore, it is unclear why risks are limited only to those that are newly observed, considering that known risks could potentially evolve into systemic risks at some point.
The systemicity of risks. The risks specific to the advanced model must be systemic. To be systemic, it must have actual or foreseeable negative effects on public health, safety, security, fundamental rights, or society at large. According to the recital in the political agreement, systemic risks include but are not limited to major accidents and sector disruptions, impact on democratic processes, public, and economic security, and the spread of illegal, false, or discriminatory content and can propagate at scale across various sectors and throughout the model’s lifecycle. Influencing factors include misuse, reliability, fairness, security, autonomy, access to tools, modalities, release strategies, removal of guardrails, and more. The recital refers to international attention given to risks from intentional misuse or control issues of AI, including lowered barriers for weapon development, enhanced cyber warfare capabilities, physical system interference, and the potential for AI models to replicate or train other models.
There is no common methodology to define systemic risk at present. Drawing from the financial sector, it largely describes the risk of ripple effects that can impact the entire ecosystem.[59] However, there is currently little experience around assessing reliably the nature of systemic risks in the tech sector.[60] By end of August 2023, designated very large online platforms and very large online search engines had to submit the first impact assessments on systemic risks under the Digital Services Act.[61] At the same time, the Commission published a related study in which it details independent authors examine an approach to systemic risk measurement in the context of disinformation and the war in Ukraine. The authors measured the severity as a function of the relationship between the qualitative assessment of the risk posed by the content in context and a quantitative measure of the reach and/or intensity of exposure of audiences to that content. It then stipulates that a risk may reach a systemic level in different ways. The higher the level of risk inherent in the content in context, the smaller the audience required to reach a systemic level. And by contrast, the lower the level of risk inherent in the content examined in context, the larger the audience required to reach a systemic level.[62] Model output differs in so far from user-generated content disseminated on online platforms as it is not directly accessible to the public.
While the measurement cannot be directly applied, it serves as an indication for how the Commission may approach the qualitative and quantitative assessment of systemic risks for foundation models.
In summary, the uncertainty over the threshold of high-impact capabilities, along with unclear benchmarks, risks included, and the systemic nature of these risks, complicates the regulatory framework. The tautologically structured definitions of high-impact capabilities, risks specific to these capabilities, and systemic risks contribute to this ambiguity, increasing the likelihood of challenges in consistent interpretation, increasing the likelihood that model providers will utilize their right to rebuttal.
III.3 Who defines the correlation between capabilities and risks?
The convergence of a pressing need for a time-sensitive resolution, along with vigorous discussions about existential risks and similarities to content moderation systemic risks in the DSA, likely played a role in shaping this situation. Over the last year, a fierce debate emerged on how risks should be classified and prioritized. A growing AI governance community focused on research on long-term and extreme or existential risks, often related and in conjunction with the research on Artificial General Intelligence (AGI) or superintelligence, has been vocal on the issue.[63] This distinct and concurrent line of research has been investigating existential threats to humanity, encompassing, but not restricted to, those arising from misaligned AGI.[64] Other researchers maintain the need to address existing systemic risks,[65] investigate critically the communication around existential risk,[66] or even argue for AI acceleration.[67]
Prominent AI laboratories such as OpenAI, Deepmind, and Anthropic have been actively expressing concerns about existential risks and emphasizing the importance of safety in their pursuit of developing artificial general intelligence (AGI).[68] OpenAI recently unveiled its new preparedness framework, outlining the organization’s methods for monitoring, assessing, forecasting, and mitigating catastrophic risks associated with progressively powerful models. Simultaneously, Anthropic introduced Version 1.0 of its Responsible Scaling Policy (RSP). These policies adopt a levels-based strategy to categorize the risk associated with different AI systems, pinpointing potential hazardous capabilities linked to each AI Safety Level (ASL), and specifying appropriate containment or deployment measures for each level. Deepmind’s research team created a taxonomy for classifying the state of the art towards AGI.[69] The taxonomy includes both performance benchmarks and generality of capabilities benchmark.[70]
Following the categorization as proposed by Deepmind, obligations might need to be extended to highly capable narrow AI, especially considering their potentially hazardous capabilities, and the question arises why such AI would not be subject to the same level of transparency obligations.
The correlation between existing/near-term harm and long-term extreme risk as elaborated by Benjamin S. Bucknall and Shiri Dori-Hacohen serves eventually as a useful connector between different risk timelines without the need to ignore the one or other.[71] The below figure illustrates a hypothesis that certain already observed systemic risks of AI (such as disinformation or hate speech on online platforms and in recommender systems) can act as existential risk factors, even in the absence of artificial general intelligence.[72]
In sum, there is an urgent need for better taxonomy for the correlation of risk classification and model capabilities as well as understanding the underlying dynamics of the benchmark and evaluation ecosystem.
IV. The role of codes and standards under the new title
In the past decade, the European Commission has increasingly utilized codes of conduct (CoC) at the EU level as a strategic tool to advance specific policy objectives in the digital realm, particularly in instances where Member States were reluctant to harmonize at the EU level, or when emerging technological developments necessitated regulatory attention. These codes range from hate speech, IP infringement, child safety and most recently disinformation.[73] They also serve diverse roles in various regulations, employing codes not merely as voluntary commitments by industry players, but more significantly as a part of co-regulatory measures. For example, in the GDPR, codes of conduct can be used to demonstrate compliance, whereas in the DSA, they are utilized as risk mitigation tools, complemented by an enforceable regulatory backstop. Many of these voluntary codes will now be transposed into codes under the DSA for very large online platforms as mitigation measures of systemic risks.
According to the GPAI compromise, Providers of GPAI models may rely on codes of practice to demonstrate compliance with the obligations in the new title, until a harmonized standard is published. The AI Office is tasked with ‘encouraging and facilitating’ the creation of codes of practice, considering international approaches.[74] Key issues include updating information in line with market and technological developments, identifying systemic risks at the Union level, and establishing measures for the assessment and management of these risks. The process invites participation from providers of general-purpose AI models, national authorities, and civil society organizations. The AI Board[75] aim to ensure these codes of practice have clear objectives and contain commitments or measures, including key performance indicators, to meet these objectives while considering the interests of all involved parties. The AI Office and the AI Board are responsible for regularly monitoring and evaluating the effectiveness of these codes, with the possibility of the Commission approving the code for Union-wide validity.
While codes serve to demonstrate compliance with the new GPAI obligations, they also allow for commitments that go beyond these obligations. This co-regulatory approach allows for the evolution of these commitments and to adapt to emerging new advancements in AI and state-of-the-art safety research. This strategy evidently gains advantages from the insights acquired through the DSA’s Code of Practice (CoP) framework experience.[76]
The identified challenges within the regulatory framework, pertaining to highly capable models, risks, systemic risks, smaller models, benchmarks, and narrow AI, might be mitigated by specifically targeting these areas in the code. In its role to define measurements and evaluations, the careful selection and balance of stakeholders is crucial, especially given the current lack of in-depth understanding of the dynamics in this field. Ultimately, the success of these codes, relevant to both designated systemic risk GPAI model providers and others, will hinge on the providers’ readiness to actively cooperate.
The reliance on self-reporting by participants as well as the need to agree on common reporting methodologies could slow down progress in the implementation and effectiveness of these codes. This potential risk is offset however by the fact that the codes of practice could encourage proactive risk management, enhancing best practices by a broader set of stakeholders. Moreover, the inclusive process of developing these codes in the context of a co-regulatory approach, involving a variety of stakeholders, could lead to more comprehensive regulations that consider a wide range of perspectives and concerns and can be quickly adapted to technology advancements.
Switching from codes of practice to CEN-CENELEC standards in AI could lead to reduced flexibility and a one-size-fits-all approach, less effective for the diverse needs of language GPAI. The lengthy process of establishing these formal standards, due to required consensus, may not keep pace with AI’s rapid evolution. This approach also risks limited stakeholder engagement,[77] possibly resulting in standards that do not fully address all concerns. While formal standards offer clarity and consistency, these challenges emphasize the importance of thoughtful transition strategies in AI regulation.
It is unclear why both harmonized standards at the European level and codes of conduct were considered necessary to be developed simultaneously or why one would replace the other. Incorporating co-regulation mechanisms such as a Code of Conduct can enhance the efficiency of standards development, particularly when implementing a risk-based regulatory approach, as safety measures progress in tandem with AI capabilities. However, in a code as foreseen in the political agreement, each signatory of the code must establish joint commitments and individual KPIs, that may be more adequate to the contextuality of foundation models, for example in view of risk mitigation measures while standards might be more appropriate for benchmarking of capabilities. The effort required from companies (and civil society) to establish these codes, only to have them potentially supplanted by concurrently developing standards, is not immediately apparent.
V. Could GPAI be better regulated in the DSA?
Safety measures in GPAI models employ methods that are like content moderation techniques, commonly used by intermediaries hosting or making third-party content publicly available. In other words, the commonality between these services lies in their shared goal of avoiding the GIGO (Garbage In, Garbage Out) effect.[78] There is a noticeable parallel to the early days of the e-commerce directive, where regulatory focus tended to prioritize IP owners concerned about their rights and future business prospects. This often resulted in comparatively less attention being given to content that could harm children, such as websites inciting suicide or training data containing images of child abuse.[79] Some have, thus, argued that the DSA is the appropriate framework for regulating foundation models.[80] The key difference between providers of models and services under the DSA is the latter’s emphasis on intermediation services. Equally important is the distinction regarding content control: the deliberate selection of training data contrasted with the relatively uncontrolled nature of user-generated content. The demarcation between various services under different regulatory regimes – including hosting services like cloud providers, online platforms such as developer platforms, model developers, and newly emerged application services – may be more ambiguous than suggested. As a result, it is recommended to maintain ongoing monitoring and potentially conduct further examinations into the interactions among these diverse services.[81] For the time being, it’s important to note that liability exemptions are solely applicable to services falling within the scope of the Digital Services Act (DSA).[82]
VLOPs that utilize GPAI for recommender systems and content moderation have naturally garnered the Commission’s attention. This focus is evident in both the code of practice on disinformation, which has established a generative AI task force, and the initial enforcement steps of the DSA, which includes VLOSEs like Bing search, Google Search, and VLOPs such as TikTok, Facebook, and others. The interplay of risk management in the DSA with the risk management in the AIA is, thus, relevant. The relevant recital stipulates that when these models are integrated into designated VLOPs or VLOSEs, they become subject to the risk management framework under the DSA.[83] The recital concludes that the corresponding obligations of the AI Act should be presumed to be fulfilled, unless significant systemic risks not covered by the DSA emerge and are identified in such models. The recital appears to intend to reduce the compliance burden, but there are several concerns. 1) The presumption that the model is developed by the service within the scope,[84] 2) the assumption that the model exhibits high-impact capabilities, and 3) the possibility that systemic risks identified in the DSA may also exist in smaller models, potentially falling outside the scope of the GPAI rules.[85] The GPAI model can, nevertheless, serve as a significant amplifier to risks within integrated platform ecosystems.[86]
However, the requirements imposed on both providers and users of specific AI systems in the AI Act, aimed at enabling the identification and disclosure of artificially generated or manipulated outputs, are especially pertinent for facilitating the efficient enforcement of the DSA. This relates to the responsibilities of VLOPs and VLOSEs to identify and address systemic risks that may arise from the dissemination of content artificially generated or manipulated. These risks include the potential for actual or foreseeable adverse impacts on democratic processes, civic discourse, and electoral procedures, particularly through the spread of disinformation.
In conclusion, there exists a notable interplay between the DSA and GPAI regulations concerning VLOPs and VLOSEs that incorporate highly capable GPAI models into their operations. It is essential to pay close attention to the clarity of the interaction to ensure effective implementation.
VI. Are the exemptions of open source related to GPAI adequate?
The provisional agreement excludes AI models that are made accessible to the public under a free and open-source license. This exemption applies when the parameters of these AI models, which include the weights, information on the model architecture, and details on model usage, are publicly available. However, there are specific obligations from which these models are not exempt, including steps to protect IP rights, i.e., putting in place a policy to respect Union copyright law to identify and a publicly available summary about the content used for training of the model. If a model is designated as entailing systemic risks, they are not exempt from the respective obligations. While the AI provides a degree of leniency for models under free and open-source licenses, this leniency is not absolute. Models entailing systemic risks are subject to regulatory scrutiny.
This approach aims to strike a balance in an issue that has sparked intense debates, with the AI safety community[87] and the open-source community[88] holding fundamentally opposing viewpoints. On one hand, it addresses safety concerns related to AI models, while on the other hand, it acknowledges the benefits of sharing knowledge within the broader community. In essence, it navigates the tension between comprehending the capabilities and limitations of model performance while simultaneously safeguarding against potential risks.
Naturally, open AI models inherently possess transparency, making general transparency rules less critical for them. However, it’s important to note that not all so-called “open” releases are truly open. A significant challenge has been the lack of a universally accepted definition to delineate what qualifies as an open model. Developers decide how to release their models, whether it’s a no release policy, gated release, APIs, staged releases, or full disclosure of all model artifacts, and decision around this often driven by navigating between safety and openness.[89] Advanced models like OpenAI’s GPT4 and Google’s PaLM2 have typically been released in a controlled manner, with access primarily through online interfaces. This gated approach is justified to avoid misuse and facilitate commercialization. In contrast, models like LLaMa have followed a more open approach. Even where committed to open research and open science,[90] providers acknowledge that some information should not be released to the public.
There are several organizations currently working to define open-source AI, among them the Open-Source Initiative (OSI),[91] the Linux Foundation,[92] and the DPGA with UNICEF.[93] Another challenge is the possible economic interests that play a significant role in keeping models close.[94] The open-source community, largely dependent on large-scale models developed by labs with access to hyperscale compute power and GPUs they do not own. This situation raises critical questions about the competitiveness of the ecosystem and the necessity for regulatory interventions to support open access, while concurrently grappling with unresolved challenges like privacy concerns.
The political agreement missed the opportunity to thoroughly consider gradient approaches to releasing AI models. The GPAI Code of conduct could further explore reasoning and responsibility of release decisions in this context in line with already ongoing international initiatives.[95]
The political agreement for advanced AI models presents several complexities. While the risk-based approach remains intact, it is not without its challenges, and there is ambiguity in identifying specific risks associated with advanced models and their correlation with capabilities. Additionally, uncertainties in benchmarking due to the contextual nature are inherent, and the need for both harmonized standards and codes of conduct concurrently, respectively the replacement of one for the other, is unclear. Despite these complexities, regulating foundation models with transparency is beneficial for the broader AI ecosystem, addressing gaps not covered by existing regulations. Exploring gradient approaches for AI model releases and addressing any remaining inconsistencies in the code of conduct for compliance can further enhance the responsible AI ecosystem and the responsible use of advanced AI models. To ensure effective implementation, clarity in the interaction between different regulations and the consideration of compliance bureaucracy for model providers remains important though.
Cover Photo : Created by DALL-E, prompt by Theodore Christakis : “Produce a contemporary and thought-provoking modern art painting that visually conveys the intricate complexities and challenges associated with regulating general-purpose AI systems in the European Union”
These statements are attributable only to the author, and their publication here does not necessarily reflect the view of the other members of the AI- Regulation Chair or any partner organizations.
This work has been partially supported by MIAI @ Grenoble Alpes, (ANR-19-P3IA-0003)
[1] Proposal for a regulation of the European Parliament and of the Council laying down harmonized rules on artificial intelligence (Artificial intelligence Act) and amending certain union legislative acts COM/2021/206 final.
[2] Commission Staff Working Document SWD/ (2021) 84 final.
[3] The term “foundation model” was coined by the Stanford Institute for Human-Centered Artificial Intelligence in 2021. Introducing the Center for Research on Foundation Models (CRFM) (stanford.edu); Foundation model – Wikipedia
[4] Jingfeng Yang a.o., Harnessing the Power of LLMs in Practice: A Survey on ChatGPT and Beyond https://arxiv.org/abs/2304.13712 , see also GitHub – Mooler0410/LLMsPracticalGuide: A curated list of practical guide resources of LLMs (LLMs Tree, Examples, Papers)
[5] A summary overview can be found here: 2023: The Year of AI. The most remarkable releases, partnerships, and legal debates (everypixel.com).
[6] See e.g., Intelligenza artificiale: il Garante blocca ChatGPT. Raccolta illecita di… – Garante Privacy
[7] See for US litigation (behind paywall): From ChatGPT to Deepfake Apps: A Running List of AI Lawsuits (thefashionlaw.com), see also https://www.wsj.com/tech/ai/new-york-times-sues-microsoft-and-openai-alleging-copyright-infringement-fd85e1c4?mod=e2tw
[8] See for example: Zoom’s updated Terms of Service permit training AI on user content without Opt-Out (stackdiary.com); Zoom responds to privacy concerns raised by AI data collection (nbcnews.com); How Zoom’s terms of service and practices apply to AI features | Zoom
[9] Including OpenAI, Microsoft, Google and recently Anthropic: https://www.anthropic.com/index/expanded-legal-protections-api-improvements
[10] AI Index Report 2023 – Artificial Intelligence Index (stanford.edu); AIAAIC Repository –.
[11] Investigation Finds AI Image Generation Models Trained on Child Abuse | FSI (stanford.edu).
[12] See for example GPT-4 Technical Report, arXiv:submit/4812508 [cs.CL] 27 Mar 2023, gpt-4.pdf (openai.com).
[13] See for example: a Taxonomy and Analysis of Societal-Scale Risks from AI; [2306.06924] TASRA: a Taxonomy and Analysis of Societal-Scale Risks from AI (arxiv.org); also Yoshua Bengio, FAQ on Catastrophic AI Risks FAQ on Catastrophic AI Risks – Yoshua Bengio
[14] See Romera-Paredes, B., Barekatain, M., Novikov, A. et al. Mathematical discoveries from program search with large language models. Nature (2023). https://doi.org/10.1038/s41586-023-06924-6.
[15] See overviews, IAPP Research and Insights, Global AI Legislation Tracker, Last updated 25 Aug. 2023, global_ai_legislation_tracker.pdf (iapp.org); also The context – OECD.AI.
[16] See Elliot Jones, 17 July 2023, Explainer: What is a foundation model? | Ada Lovelace Institute
[17] By the end of the Spanish presidency, no text has been available and technical discussions continue until 9 February. Then COREPER will formerly adopt the text before the Parliament can approve it, at the latest on 25 April which is the last session before election.
[18] Used synonymously for foundation models.
[19] Not publicly available.
[20] As of writing, no text has been made public yet. The analysis is based on a compromise proposal distributed on 7 December by the Spanish presidency (leaked by contexte https://www.contexte.com/medias/pdf/medias-documents/2023/12/aia-draft-deal-gpai-82bc6dbbbf73463986ec4b9f45e203f0.pdf).
[21] Regulation (EU) 2022/2065 of the European Parliament and of the Council of 19 October 2022 on a Single Market For Digital Services and amending Directive 2000/31/EC (Digital Services Act).
[22] Regulation (EU) 2022/1925 of the European Parliament and of the Council of 14 September 2022 on contestable and fair markets in the digital sector and amending Directives (EU) 2019/1937 and (EU) 2020/1828 (Digital Markets Act).
[23] FLOPs, or Floating-Point Operations Per Second, measure a computer’s processing speed, focusing on its ability to perform floating-point calculations. High FLOPs suggest a system’s potential to handle complex and large-scale deep learning tasks, which often requires significant computational resources essential for advanced foundation models in AI. More FLOPs mean better handling of extensive data and intricate computations providing a useful measure of computational power.
[24] The efficiency and architecture of computational hardware is constantly improving, making pure FLOPs a less precise measure of actual performance capabilities. In conclusion, their relevance and precision as a sole performance indicator may diminish with advancing technology and evolving computational paradigms.
[25] Medium, Greg Broadhead, Aug 25, 2023, A Brief Guide To LLM Numbers: Parameter Count vs. Training Size
[26] Threshold not defined in the version of the agreement at hand.
[27] The documentation for GPAI models, as example, include a general overview, including its intended tasks, types of compatible AI systems, usage policies, release date, marketing strategy, and basic model details like architecture, dataset, and licensing. Additionally, it should offer a detailed technical breakdown, encompassing the model’s design specifications, input/output formats, comprehensive training data information, and computational resources used in training, such as energy consumption and operational specifics.
[28] De Gregorio, Giovanni and Dunn, Pietro, The European Risk-Based Approaches: Connecting Constitutional Dots in the Digital Age (March 31, 2022). 59(2) Common Market Law Review 2022, 473-500, Available at SSRN: https://ssrn.com/abstract=4071437 or http://dx.doi.org/10.2139/ssrn.4071437
[29] As opposed to the DSA and the GDPR, supra.
[30] Recital 60 of the proposal for a regulation of the European Parliament and the Council laying down harmonized rules on artificial intelligence (artificial intelligence act) and amending certain Union legislative acts, COM(2021) 206 final.
[31] See table 3 below.
[32] Progress report of the Slovenian presidency 22 November 2021; ST-13802-2021-REV-1_en.pdf (europa.eu).
[33] Consolidated version of the Council amendments (15 June 2022); ST-10069-2022-INIT_x.pdf (europa.eu).
[34] General approach 22 November 2022; ST-14954-2022-INIT_en.pdf (europa.eu).
[36] https://www.europarl.europa.eu/doceo/document/TA-9-2023-0236_EN.html.
[37] See Zalando v Commission Case T-348/23; Amazon Services Europe v Commission (Case T-367/23)
[38] The Commission opened four market investigations to further assess Microsoft’s and Apple’s rebuttal that their core platform services do not qualify as gateways (Bing, Edge, Microsoft Advertising, Apple iMessage), Commission designates six gatekeepers under the Digital Markets Act – European Commission (europa.eu).
[39] A political deal was achieved in December 2023 for the Product Liability directive, https://www.europarl.europa.eu/legislative-train/theme-a-europe-fit-for-the-digital-age/file-new-product-liability-directive; the AI Liability directive is currently on halt.
[40] Techcrunch, Natasha Lomas, 11 December 2023, https://techcrunch.com/2023/12/11/eu-ai-act-gpai-rules-evolve/.
[41] See also Frontier Model Forum.
[42] The Shape of Code » Growth in FLOPs used to train ML models (shape-of-code.com)
[43] EU AI Act – Compliance Analysis General-Purpose AI – Models in Focus, The Future Society.
[44] Compute trends across three areas of machine learning 2202.05924.pdf (arxiv.org)
[45] Frontier models: managing emerging risks to public safety 2307.03718.pdf (arxiv.org)
[46] Scaling Laws for Neural Language Models 2001.08361.pdf (arxiv.org)
[47] LLaMA: Open and Efficient Foundation Language Models; 2302.13971.pdf (arxiv.org).
[48] [2203.15556] Training Compute-Optimal Large Language Models (arxiv.org)
[49] An Automatic Evaluator for Instruction-following Language Models: https://tatsu-lab.github.io/alpaca_eval/; Chatbot Arena ⚔️ : Benchmarking LLMs in the Wild: https://arena.lmsys.org/; HuggingFaceH4/open_llm_leaderboard https://huggingface.co/spaces/HuggingFaceH4/open_llm_leaderboard; see also The LLM Index: The Large Language Model (LLM) Index | Sapling and “Judging LLM-as-a-Judge with MT-Bench and Chatbot Arena”
[50] Holistic Evaluation of Language Models (HELM) (stanford.edu);
[51] Holistic Evaluation of Language Models, Center for Research on Foundation Models (CRFM) at the Stanford Institute for Human-Centered Artificial Intelligence (HAI), https://crfm.stanford.edu/helm/v1.0, 2211.09110.pdf (arxiv.org).
[52] Table from Holistic Evaluation of Language Models, Center for Research on Foundation Models (CRFM) at the Stanford Institute for Human-Centered Artificial Intelligence (HAI), https://crfm.stanford.edu/helm/v1.0, 2211.09110.pdf (arxiv.org)
[53] See e.g., BoolQ: Exploring the Surprising Difficulty of Natural Yes/No Questions 1905.10044.pdf (arxiv.org), Measuring Massive Multitask Language Understanding 1905.10044.pdf (arxiv.org).
[54] Martínez-Plumed, F., Barredo, P., hÉigeartaigh, S.Ó. et al. Research community dynamics behind popular AI benchmarks. Nat Mach Intell 3, 581–589 (2021). https://doi.org/10.1038/s42256-021-00339-6
[55] Structured_Access_for_Third-Party_Research.pdf (governance.ai).
[56] See OpenAI GPT-4 Technical Report, arXiv:submit/4812508 [cs.CL] 27 Mar 2023, gpt-4.pdf (openai.com).
[57]OpenAI March 2023 gpt-4-system-card.pdf (openai.com).
[58] See OpenAI GPT-4 Technical Report, arXiv:submit/4812508 [cs.CL] 27 Mar 2023, gpt-4.pdf (openai.com).
[59] What Is Systemic Risk? Definition in Banking, Causes and Examples (investopedia.com)
[60] Elements of effective risk assessment under the DSA; CERRE-DSA-Systemic-Risk-Report.pdf
[61] See Designation decisions for the first set of Very Large Online Platforms (VLOPs) and Very Large Online Search Engines (VLOSEs) | Shaping Europe’s digital future (europa.eu).
[62] European Commission, Directorate-General for Communications Networks, Content and Technology, Digital Services Act – Application of the risk management framework to Russian disinformation campaigns, Publications Office of the European Union, 2023, https://data.europa.eu/doi/10.2759/764631.
[63] The term is not defined in the AI Act and is a controversial concept in computing research. Generally described as at least as capable as humans at most tasks. See e.g., the Machine Intelligence Research Institute (MIRI)’s background claims https://intelligence.org/2015/07/24/four-background-claims/
[64] Prominent institutes include the the Machine Intelligence Research Institute (MIRI), Future of Humanity Institute (FHI); Centre for the Study of Existential Risk (CSER), dedicated to the study and mitigation of risks that could lead to human extinction, including AI risks; Stanford Institute for Human-Centered Artificial Intelligence (HAI): includes the study of ethical and societal impacts of AI, encompassing existential risks; the Future of Life Institute (FLI): works to mitigate existential risks facing humanity, particularly those arising from advanced AI technologies; the Machine Intelligence Research Institute (MIRI). A list of additional groups can be found here Ultimate Guide to “AI Existential Risk” Ecosystem (aipanic.news)
[65] DAIR, Statement from the listed authors of Stochastic Parrots on the “AI pause” letter | DAIR (dair-institute.org); see also 3442188.3445922.pdf (acm.org); On the Dangers of Stochastic Parrots | Proceedings of the 2021 ACM Conference on Fairness, Accountability, and Transparency; Timnit Gebru, Eugenics and the Promise of Utopia through AGI.
[66] Nirit Weiss-Blatt on substack: Ultimate guide to existential risk eco-system, the AI panic campaign.
[67] What is e/acc? (perplexity.ai).
[68] Research (openai.com); DeepMind AGI; Research \ Anthropic
[69] Levels of AGI: Operationalizing Progress on the Path to AGI; [2311.02462] Levels of AGI: Operationalizing Progress on the Path to AGI (arxiv.org)
[70] Levels of AGI: Operationalizing Progress on the Path to AGI; [2311.02462] Levels of AGI: Operationalizing Progress on the Path to AGI (arxiv.org)
[71] Benjamin S. Bucknall and Shiri Dori-Hacohen: Current and near-term AI as potential existential risk factor 2209.10604.pdf (arxiv.org)
[72] Benjamin S. Bucknall and Shiri Dori-Hacohen: Current and near-term AI as potential existential risk factor 2209.10604.pdf (arxiv.org)
[73] The 2022 Code of Practice on Disinformation | Shaping Europe’s digital future (europa.eu); The EU Code of conduct on countering illegal hate speech online – European Commission (europa.eu); A European strategy for a better internet for kids (BIK+) | Shaping Europe’s digital future (europa.eu); Memorandum of understanding on online advertising and IPR – European Commission (europa.eu)
[74] E.g., the Hiroshima Process International Code of Conduct for Organizations Developing Advanced AI Systems, 100573473.pdf (mofa.go.jp)
[75] The AI Board, which would comprise member states’ representatives, will remain as a coordination platform and an advisory body to the Commission and will give an important role to Member States on the implementation of the regulation, including the design of codes of practice for foundation models. Finally, an advisory forum for stakeholders, such as industry representatives, SMEs, start-ups, civil society, and academia, will be set up to provide technical expertise to the AI Board.
[76] The 2022 Code of Practice on Disinformation | Shaping Europe’s digital future (europa.eu)
[77] Veale, Michael and Zuiderveen Borgesius, Frederik, Demystifying the Draft EU Artificial Intelligence Act (July 31, 2021). Computer Law Review International (2021) 22(4) 97-112, Available at SSRN: https://ssrn.com/abstract=3896852
[78] Garbage in, garbage out – Wikipedia.
[79] See for example Identifying and Eliminating CSAM in Generative ML Training Data and Models, Identifying and Eliminating CSAM in Generative ML Training Data and Models, David Thiel, Stanford Internet Observatory, December 23, 2023 https://stacks.stanford.edu/file/druid:kh752sm9123/ml_training_data_csam_report-2023-12-23.pdf
[80] Botero Arcila, Beatriz, Is it a Platform? Is it a Search Engine? It’s Chat GPT! The European Liability Regime for Large Language Models (August 12, 2023). Journal of Free Speech Law, Vol. 3, Issue 2, 2023, Available at SSRN: https://ssrn.com/abstract=4539452
[81] Elkin-Koren, Niva and Perel (Filmar), Maayan, Algorithmic Governance by Online Intermediaries (July 13, 2018). Oxford Handbook of International Economic Governance and Market Regulation (Eric Brousseau, Jean-Michel Glachant, & Jérôme Sgard Eds.) (Oxford University Press, 2018, Forthcoming)., Available at SSRN: https://ssrn.com/abstract=3213355
[82] Regulation (EU) 2022/2065
[83] The recital further explains that “Within this framework, providers of very large online platforms and very large search engines are obliged to assess potential systemic risks stemming from the design, functioning and use of their services, including how the design of algorithmic systems used in the service may contribute to such risks, as well as systemic risks stemming from potential misuses. Those providers are also obliged to take appropriate mitigating measures in observance of fundamental rights.”
[84] See respectively a parliamentary question by MEP Schaldemose, https://www.europarl.europa.eu/doceo/document/E-9-2023-003694_EN.html submitted on 15 December 2023: “AI Are AI systems regulated under the DSA when they are used as a service on a platform?”
[85] Schwemer, Sebastian Felix, Recommender Systems in the EU: from Responsibility to Regulation? (September 13, 2021). FAccTRec Workshop ’21, September 27–October 1, 2021, Amsterdam, Netherlands, Available at SSRN: https://ssrn.com/abstract=3923003
[86] Elements of effective risk assessment under the DSA; CERRE-DSA-Systemic-Risk-Report.pdf
[87] https://www.governance.ai/research-paper/open-sourcing-highly-capable-foundation-models
[88] supporting_OS_in_the_AIAct.pdf (eleuther.ai)
[89] Workshop on Responsible and Open Foundation Models, https://www.youtube.com/watch?v=75OBTMu5UEc&t=3612s, summary here: https://hai.stanford.edu/news/how-promote-responsible-open-foundation-models
[90] EleutherAI: Going Beyond “Open Science” to “Science in the Open” 2210.06413.pdf (arxiv.org); Why Release a Large Language Model? | EleutherAI Blog
[91] https://opensource.org/deepdive/; current draft: https://opensource.org/deepdive/drafts/the-open-source-ai-definition-draft-v-0-0-4/
[92] https://www.linuxfoundation.org/research/artificial-intelligence-and-data-in-open-source
[93] https://digitalpublicgoods.net/blog/exploring-a-gradient-approach-to-the-openness-of-ai-system-components/
[94] See Dwaresh podcast, Nat Friedman – Reading ancient scrolls, open source & AI.
[95] https://digitalpublicgoods.net/blog/exploring-a-gradient-approach-to-the-openness-of-ai-system-components/