Table of Contents
Executive summary
Significant progress has been made in recent years in the field of image processing, particularly in facial recognition. The number of deployments and experiments of this type of system is rapidly increasing. Most applications are motivated either by security or by commercial considerations. However, there are different opinions regarding the use of these systems, particularly in the public space. Considering the lack of consensus on a technology that can have a significant impact on society, many organizations have alerted public opinion and called for a public debate on this topic. We believe that such a debate is indeed necessary. However, for such a debate to be productive, it is essential to ensure that arguments can be expressed and confronted in a rigorous way. In particular, it is critical to avoid, as much as possible, preconceptions and to distinguish established facts from assumptions or opinions.
The purpose of this document is precisely to set the terms of the debate on a solid basis. Our aim is not to take a position on facial recognition in general or to provide an exhaustive review of its applications, but rather to propose some elements of methodology for the analysis of its impacts, illustrated by some examples. The importance of conducting impact assessments of facial recognition technologies is underlined by institutions such as the European Commission and the European Union Agency for Fundamental Rights (FRA) but there is still a lack of methodology to put them into practice.
As facial recognition applications are very different, it is necessary to precisely analyse the potential impacts of each system, taking into account all its features and the context of its deployment. However, this case-by-case analysis should not overlook the more “systemic” risk related to a potential generalization of the reliance on facial recognition in our societies. This global risk must also be analysed and debated because it may justify, according to some people, a total or partial ban of facial recognition.
In this document, we first present a brief overview of the applications of facial recognition before discussing the reasons why this technology raises specific issues and discuss the risks associated with a possible generalization of its use. We then propose an incremental, comparative and rigorous approach to analyse the impacts of a facial recognition system.
- Our approach is incremental in the sense that it leads to successive analyses of the impacts related to (1) the purpose of the system, (2) the means chosen to achieve this purpose, (3) the use of facial recognition to achieve these means and finally (4) the specific implementation of facial recognition in the system.
- It is comparative in the sense that it advocates an assessment of the benefits and the risks in comparison with other possible options at each of the four steps.
- Finally, it is rigorous in the sense that it requires to define the status of each argument: for example, claims already corroborated by experimental results or studies validated by the scientific community; claims that are not supported by sufficient studies but could be subject to validation; and claims that are based on subjective or political positions that are not amenable to experimental evaluation.
We also stress the importance of accountability, which is an essential component to enhance trust in facial recognition systems. Indeed, once images are captured, recorded and potentially analysed, it is impossible to provide an absolute guarantee that the system cannot be misused or produce unexpected effects. It is therefore of prime importance to put in place technical, legal and organisational measures to ensure that entities exploiting these systems are accountable for their use. To be effective, such measures must be monitored by a competent independent body in a situation to provide all stakeholders with some visibility and elements of trust on the actual use of the system.
In cases where prior field experiments are deemed necessary, these experiments must also be subject to an impact analysis. In addition, to be really useful and legitimate, they must follow a rigorous protocol. In particular, their objectives, the conditions under which they will be conducted, the underlying assumptions, and the evaluation criteria should be defined precisely.
In conclusion, we stress the need to advance the state of the art to improve the trust that can be placed in facial recognition systems and propose several concrete actions in this direction. We also stress that fact that while the current focus on automated facial recognition is important and desirable, we should not forget that it is only one technology amongst many others to identify and profile users. Facial recognition should therefore be analysed in the broader context of tracking and profiling technologies.
We hope that the methodology proposed in this report can be useful at two levels of discussion:
- First, in the context of the general debate that should take place about the deployment of facial recognition in our societies. This debate must consider all the potential impacts of this technology and must be conducted in an open manner, without excluding the possibility of a total or partial ban or authorizations under certain restrictions or conditions.
- Second, for the case-by-case analysis of each project (in the event that the previous debate would not lead to an overall ban).
In addition, we believe that it can also be applied, mutatis mutandis, to any AI or algorithmic system. This generalization will be the subject of future work.
1. Introduction
Significant progress has been made in recent years in the field of image processing, particularly in facial recognition. The number of deployments and experiments of this type of system is rapidly increasing. Most applications are motivated by security (identification and tracking of criminals, detection of suspicious or behaviours, etc.), safety (detection of dangerous behaviours, search for lost persons, etc.) or commercial considerations (analysis of customer behaviour, etc.).
In a nutshell, facial recognition consists of establishing a link between two images representing faces. It can be applied in different ways, including authentication – i.e. verification of identity, identification – i.e. establishment of identity, or tracking – i.e. tracking a face on several video images. There are diverging opinions on the use of these technologies, particularly in public spaces. Some, such as the mayor of the city of Nice in France, complain about the fact that they cannot use solutions that would make it possible to identify individuals that are recorded in databases of wanted or dangerous people in order to avoid terrorist attacks [1]:
“I have the software that would allow me to apply facial recognition and identify individuals wherever they are in the city. Why should this be banned? Do we want to take the risk of people dying in the name of individual freedoms, when we have the technologies to avoid it?”
In the same vein, James O’Neill, New York Police Commissioner, highlights the usefulness of facial recognition to track suspects and concludes that “it would be an injustice to the people we serve if we policed our 21st century city without using 21st century technology” [2].
Others, such as the CNIL, warn against this logic of mass surveillance and are concerned about the disproportionate threats to individual freedoms posed by these technologies [3]:
“But these devices, which are sometimes linked to big data technologies, raise important issues with regard to individual rights and freedoms of citizens. The feeling of increased surveillance, the increased, automated and potentially large-scale use of personal data, including sensitive (biometric) data, the restriction of the freedom to come and go anonymously, and the predictive nature of its technologies are all essential issues for the proper functioning of our democratic society.”
Several American cities have followed the example set by San Francisco and decided to ban the use of facial recognition by their municipal services, including their police forces. For its part, Axon’s ethics committee recommended that police body cameras should not be equipped with facial recognition functionality [4]. Others are calling for a complete ban of the deployment of these techniques [5]. We are therefore in a contrasting situation today with, on the one hand, providers of facial recognition technology who often succeed in convincing political decision-makers (and citizens) of their effectiveness in improving public security and safety and, on the other hand, regulatory authorities, NGOs or researchers sounding the alarm.
Considering the lack of consensus on a technology that can have a significant impact on society, many organizations, including public bodies (CNIL, AINow, etc.), NGOs (ACLU, EFF, La Quadrature du Net, etc.) and private companies (Google, Microsoft, etc.) have alerted public opinion and called for a public debate on facial recognition. For example, the CNIL “urgently calls for a democratic debate on this topic, and for the legislator and then the regulatory authorities to take up these issues so that appropriate frameworks can be defined, seeking the right balance between security requirements, particularly in public spaces, and the preservation of everyone’s rights and freedoms” [6].
We believe that such a debate is indeed necessary. However, in order to be really productive, it is essential to ensure that arguments can be expressed and confronted in a rigorous way. In particular, it is critical to avoid, as much as possible, preconceptions and to distinguish established facts from assumptions or opinions. Indeed, arguments on this matter often mix different levels of discourse – some of them concern facial recognition in general, others concern application contexts or particular choices of implementation – and do not always make the distinction between objective facts and subjective statements or political opinions.
As facial recognition applications are very different, it is necessary to precisely analyse the potential impacts of each system, taking into account all its features and the context of its deployment. For example, the use of facial recognition for a digital identity application, such as the ALICEM [7] project in France, introduces risks that are, by nature, very different from those resulting from a system aimed at securing public space, such as the systems experimented in UK or tested in the city of Nice. These applications do not have the same purposes, do not use the same forms of facial recognition and their implementations are very different. However, this case-by-case analysis should not overlook the more “systemic” risk related to a potential generalization of the reliance on facial recognition in our societies. This global risk must also be analysed and debated because some believe that it may justify a total ban of facial recognition or its ban on public places. Moreover, a facial recognition application should not be considered exclusively as a technical object but rather as a socio-technical system, taking into account all its facets, including its economic, social and psychological dimensions [8].
The CNIL recently published a report on facial recognition presenting some technical, legal and ethical issues that must be taken into account in this debate [9]. The CNIL report discusses in particular the legal framework within which experiments and deployments of facial recognition systems may be carried out. The present document is complementary to the CNIL report in that it proposes a systematic approach for analysing the impacts of facial recognition applications. Our main objective is to help set the terms of the debate on a solid basis. The aim here is therefore not to take a position on facial recognition in general or to provide an exhaustive review of its applications, but to propose a methodology for the analysis of its impacts, illustrated by some examples. The examples selected here [10] concern essentially the use of facial recognition in the context of public services, but the approach is general and can be applied equally to other types of applications.
We hope that the methodology proposed in this report can be useful at two levels of discussion:
- First, for the general debate that should be launched about the deployment of facial recognition in our societies. This debate must consider all the potential impacts of this technology and must be conducted in an open manner, without excluding the possibility of a total ban or authorization under certain restrictions or conditions.
- Second, for the case-by-case analysis of each facial recognition project or system (in the event that the previous debate would not lead to an overall ban).
Before presenting our methodology in Section 3, we summarize in Section 2 the different types of facial recognition systems, as well as the issues related to their deployment. We conclude with perspectives and some concrete proposals in Section 4. Interested readers may also find in Appendix 1 a classification of the different applications of facial recognition and in Appendix 2 an example showing how ethical matrices can be used to synthesize the impacts identified during the analysis.
2. Facial Recognition: What are we talking about?
The expression “facial recognition” is very generic and covers a wide range of applications. We present a brief overview of these applications (Section 2.1) before reviewing the reasons that make facial recognition a particularly sensitive topic (Section 2.2), in particular because of the risk of its progressive generalization in the future (Section 2.3).
2.1 The different types of facial recognition
In this report, we are particularly interested in facial recognition techniques, i.e. applications that, roughly speaking, consist of establishing a proximity link between two images representing faces. However, our approach can be applied to other types of systems, in particular to behaviour analysis techniques. More precisely, facial recognition uses algorithms that analyse the faces in photographs or videos to extract a set of distinctive features. These physical attributes, such as the distance between the eyes or the shape of the chin, are then coded as mathematical representations, commonly referred to as “templates”. Templates, which include only the most significant features of the faces, are either stored or compared to those contained in a database.
Most facial recognition systems consist of two main components: an image processing component and one or several databases containing pre-registered information (such as templates or images). The image processing component captures and processes images. The database usually contains the information, for example the face images of authorised individuals that are used as a reference to compare with live images (captured “on the fly”). This database can be either centralized or distributed. It can be controlled by a single or several entities. In the case where it is distributed on devices, such as on dentity cards or mobile phones, it can even remain under the control of the individuals. As we will discuss in this report, the risks associated with a facial recognition system depend on the choices made for both the image processing and the database components. It is therefore important to consider the whole system when analysing the risks associated with a facial recognition system, and not to focus exclusively on the image processing component as it is often the case.
As far as the image processing component is concerned, it is useful to categorise facial recognition according to two parameters (X,Y): the number of persons represented [11] in the images captured on the fly (X) and the number of people who are part of the database (Y):
- Authentication (1:1) is used to verify an identity. This is what the owner of a mobile phone with facial recognition functionality does to unlock the device: the photograph of his face is compared to the one stored on the device.
- Identification (1:N) is used to recover the identity of a person from a database. This user may be a suspect whose photograph is to be compared with those contained in a database of wanted persons. It is also possible to generalize to a non-targeted search (N:M), such as the systematic analysis of video surveillance images to locate faces contained in a database of wanted persons. Another, more extreme variation would be the association of an identity to each face appearing on images, for example via augmented reality glasses (the number of people concerned in the database could then be the entire population).
- Tracking (1:0) can be applied to compare several images, for example captured in vivo, to track the whereabouts of a pickpocket or a suspect on video surveillance images. In this case, it is not necessary to have a database of photographs.
In addition, facial recognition can be used either “in real time”, i.e. when capturing images [12], or “a posteriori” [13], i.e. on recorded images. The second use corresponds to the scenario where images from a video surveillance system are used, for example after a crime, to identify potential perpetrators [14]. The interested reader can find in Annex 1 a more complete categorisation showing the diversity of facial recognition application modalities.
The risks induced by the different uses are of course different and more or less severe. For example, systems that use centralized databases generally introduce additional risks (including hacking or leakage risks). Similarly, the risks increase with the number of people involved (i.e. the size of the database or the number of persons whose faces are captured in vivo). Some applications may therefore be more acceptable than others. For example, Wojciech Wiewiórowski, the European Data Protection Supervisor, distinguishes between the use of facial recognition for authentication, including border controls, which he considers reasonable, and its use for identification or search in the public space, which he considers much more questionable [15].
2.2 Why is facial recognition so concerning?
The concerns raised by the development of facial recognition stem from a combination of features that may lead to serious threats to civil liberties:
- It is a biometric technique, which uses features of the human body that a person cannot change, at least not easily, unlike digital attributes (mobile phone identifiers, cookies, etc.). The sensitive nature of biometric data is recognized by law. For example, the General Data Protection Regulation (GDPR) [16] prohibits the processing of biometric data for identification purposes unless one of the ten exceptions listed in Article 9(2) can be invoked. In addition, the Charter of Fundamental Rights of the European Union, which enshrines the respect for privacy and the protection of personal data in Articles 7 and 8, specifies that “any on the exercise of the rights and freedoms recognised by this Charter must be provided for by law and respect the essence of those rights and freedoms. Subject to the principle of proportionality, limitations may be made only if they are necessary and genuinely meet objectives of general interest recognised by the Union or the need to protect the rights and freedoms of others” (Article 52).
- Unlike other biometric features, such as fingerprints or genetic data, facial images can be captured without a person’s knowledge, remotely, without contact, and in a very cost-effective way. In fact, they are already collected in large quantities, in particular through the many video surveillance cameras that are deployed in most countries. The face is also the most visible part of the body, the most difficult to dissimulate.
- Unlike other biometric features, which require an enrolment phase, i.e. the initial capture of biometric information, facial images are already available on a large scale: many public or private actors may have a large quantity of images that have been collected for other purposes or that are accessible via the Internet [17] For example, half of the U.S. adult citizens have pictures of their faces included in databases (including driver’s license databases) that are accessible by the FBI [18]. In France, any citizen applying for an identity card is now registered, with his or her photograph, in a database called TES [19].
- Consent, which is a common legal basis for the collection of personal data, is very difficult to implement for facial recognition in public spaces [20]. Signs indicating of the presence of video surveillance cameras are not effective and do not allow for a really free and informed choice since the only alternative to consent is to remain outside the area covered by the cameras.
- Despite the great progress that has been made in recent years, particularly thanks to the development of deep learning and the possibility of exploiting large image databases, the performance of most facial recognition systems are quite limited. Depending on the shooting conditions and the context of their use, they can have very high rates of false positives (people wrongly recognized) and/or false negatives (people wrongly not recognized) and theses rates may vary according to categories of population. Some systems have better results on people with white skin than on people with dark skin, on men than on women or on adults than on adolescents. These biases lead to different types of discriminations against certain populations, which are now well documented [21].
- Facial recognition systems rely on databases that contains very sensitive information and require very rigorous management procedures. Many facial recognition risks are related to the management, integrity or confidentiality of these databases.
These observations have led Woodrow Hartzog, Professor of Law at Samford University (USA), to describe facial recognition as “the most uniquely dangerous surveillance mechanism ever invented” [22]. Similarly, the British association Liberty describes it as “arsenic in the water of democracy” [23]. So far, these concerns have failed to prevent the proliferation of initiatives, often in an unclear legal context (or even unlawfully), fuelled by the bloated promises made by facial recognition promoters. The most common marketing arguments are the improvement of public safety, in particular through the analysis of video surveillance images, and gains on time time, for example for border controls. Another popular argument is that facial recognition can simplify everyday lives, for example by allowing people to enter a room without having to show a badge, or to leave a store with their purchases without having to go through a checkout. This increasing use of facial recognition devices for convenience gives rise to the deeper fear of a progressive generalization of the reliance on this technology, which could become unavoidable and have major consequences for our societies.
2.3 Risks related to a possible generalization of facial recognition
The risk assessment of any particular facial recognition system must also include a global analysis of the effect of the deployment of this technology on our societies. This preliminary step is necessary because, even if the use of specific systems may seem appropriately regulated at a given point of time, the possibility of extending their use at a later date can never be completely ruled out. Such extensions can take place in many different ways, for example by using data collected on social networks [24] or databases originally set up for different purposes, as discussed above with the FBI, or by using a database beyond the allowed purpose [25]. Extensions may also result from the introduction of new functionalities to an existing system, for example by extending facial recognition used for passport control to payments in an airport and then throughout the whole city, or by integrating the detection of suspicious behaviours into an existing video surveillance system [26]. It may be the case that some of these extensions will not be accepted by the populations, but experience shows that when they are carried out in a very progressive way and presented as natural evolutions, they do not encounter major opposition. Furthermore, in general, when humans have to balance immediate and identifiable benefits, however minor, against potentially serious but uncertain and vague harms, the trend is generally to disregard the latter.
If we assume that no technical, legal or social obstacles could prevent the current trend towards an increasingly widespread use of facial recognition, it is important to analyse the possible consequences of such a generalisation on society. The following questions can be asked in particular:
- What are the possible effects on society of a widespread use of facial recognition?
- Are these effects desirable or not?
- Can negative effects be prevented or reduced, and positive effects enhanced or amplified?
- In view of the answers to the previous questions, should the use of facial recognition technologies be banned (partially or totally)?
- If not banned, what are the main principles to be applied to the development and deployment of facial recognition? What technical, legal and/or organisational measures can be put in place to limit the risks of uncontrolled expansion?
In this respect, it is important to take into account the fact that a large number of video surveillance cameras are already deployed in public spaces. At present, these images are either viewed live by supervisory agents or a posteriori by investigators [27]. The analysis of the impacts of facial recognition in this context must therefore compare the current situation (supervision and recognition by human agents) with an implementation based on automatic recognition. The advantages and disadvantages of each option must be weighed, considering the protective measures that can be put in place in both cases and without underestimating the risks associated with the current situation. Indeed, several studies have shown the serious abuses resulting from human supervision [28]. The replacement of certain tasks by automatic processing could make it possible to limit these deviations, or at least make them more traceable, if sufficient protective measures are implemented. Conversely, if the impact analysis leads to the conclusion that any use of these video surveillance images represents a disproportionate risk, whether the analyses are carried out by human operators or by a facial recognition system, then it is the use of video surveillance itself that should be challenged.
The states are not the only source of risk in terms of surveillance. Interpersonal surveillance, sometimes referred to as “sousveillance” [29], should not be overlooked. According to anthropologist Judith Donath [30], walking with a covered face in the public space is not always well accepted and creates a feeling of insecurity, which has led some countries to regulate on this matter. According to her, the same could happen tomorrow with persons who will prefer not to be identifiable in the streets, for example through augmented reality glasses. We could thus evolve in a city where everyone would have a form of knowledge of everyone, where we would never meet a perfect “stranger”. This would certainly be a major evolution in the way we conceive the public space, with multiple consequences on social relations. Some could be considered positive, such as feeling safer, or even getting to know people they have never met, based on common tastes or friends revealed by their profiles. Others would be negative, such as the impossibility of moving anonymously in the public space, the risk of stigmatization based on the information contained in the profiles (criminal convictions, country of origin, religion, sexual preferences, etc.), the new forms of insecurity to which these profiles could give rise, the conformism induced by this total transparency, etc.
3. Incremental impact analysis
This section presents a risk-analysis methodology for facial recognition systems. We provide an overview of our approach (Section 3.1) before describing each step with some examples (Section 3.2).
3.1 A four-phase process
The approach has three main characteristics: it is incremental, comparative and rigorous. We discuss each of these aspects in turn.
Incremental approach: Our analysis framework distinguishes four levels (or phases) of analysis:
- The purpose of the system. The objective of this phase is to analyse the declared purpose of the system, independently of the means used to realize it (in particular through facial recognition). This critical analysis can question the purpose or make it more precise. Examples of purposes could be “to prevent a terrorist from committing an attack on a train” or “to ensure that only authorized persons can enter a building”.
- The means to achieve the purpose. The objective of this phase is to analyse and question the means adopted to achieve the goal, without reference to any particular technology such as facial recognition. The means describes the strategy adopted to achieve the purpose, independently of a particular implementation, by a computer system and/or human operators. For example, a hypothetical way to accomplish the purpose of “preventing a terrorist from committing an attack on a train” could be to “control, when accessing the platform, that (1) the face of the traveller corresponds to a photograph associated with a valid ticket in the system, and (2) this photograph or the identity of the traveller is not contained in a police database”. The aim is either to question this means, to improve it or to propose alternatives. In the previous train security example, as detailed in section 3.2.2, it could be argued that the proposed means is not appropriate for the stated purpose because access control is not sufficient to prevent terrorist attacks.
- The use of facial recognition technology to achieve the means. The objective of this phase is to question the use of facial recognition to achieve the means (without reference to a particular implementation of the technology). In the example of the train mentioned above, facial recognition could take place in the gateways used by travellers to get access to the platforms. When the traveller arrives at a gateway, a photograph is automatically taken and a face is extracted. This extraction is then used to automatically verify in a database that there is a reservation for a person corresponding to this face and this person does not appear in the police database. It is assumed at this stage that facial recognition is implemented in a “perfect” way. In particular, it is assumed to be accurate, free of bias and secure. Furthermore, the associated databases are assumed to be managed in a secure way. The issues addressed in this phase are therefore more theoretical than experimental by nature: they concern the inherent benefits and risks of facial recognition, independently of its actual implementation and current technology state-of the art.
- The implementation of facial recognition in a particular system. The objective of this phase is to question the implementation of facial recognition by considering the technical details of the system and the context of its deployment. The issues addressed in this phase are therefore of a concrete nature: they concern the advantages and risks of the chosen solution and its deployment.
These four levels, which constitute the four stages of the impact analysis of facial recognition systems, are represented in Figure 1. Each of these levels must be precisely described and analysed, taking into account the shor and long-term benefits and risks for all stakeholders, in particular for the people who may be affected by the deployment of the system. Section 3.2 provides more details on how to conduct these analyses and Annex 2 describes an example of the use of ethical matrices in this context. It should also be emphasized that, even if this possibility is not represented in Figure 1, this incremental process may actually give rise to iterations when the analysis leads to a revision of certain choices.
Figure 1 also includes a fifth step, a Data Protection Impact Assessment (DPIA), which is required by the GDPR and the Law Enforcement Directive for applications processing biometric data. It should be noted that, even if this is not its primary objective, the approach proposed in this note may be used to feed a DPIA. In particular, it includes, as required by Article 35 of the GDPR, “a systematic description of the envisaged processing operations and the purposes of the processing” and “an assessment of the necessity and proportionality of the processing operations in relation to the purposes”. However, the objective of our method is different since it is not only about “an assessment of the risks to the rights and freedoms of data subjects” and its goal is not focused on the protection of personal data as provided for in the GDPR. Our approach consists in assessing and comparing the benefits and risks at the four levels mentioned above, considering the overall impacts, positive and negative, on society. Our analysis goes beyond personal data processing and covers ethical issues such as fairness or consequences in terms of well-being [31]. We also stress the fact that the impact analysis should, at each stage (purpose, means, use of facial recognition and its implementation), question the proposed choices and potentially consider their rejection or the adoption of alternative solutions. Conversely, we do not consider the legal dimension here, such as the legal basis for the processing, which should be part of the DPIA.
The decomposition of the analysis into four stages, as suggested here, is useful to identify the actual causes of the impacts considered and to substantiate conflicting arguments more precisely. Indeed, there may be situations where the purpose itself is questionable: this could be the case, for example, of a project aimed at assigning a level of trust to each citizen, as in the social scoring system currently being deployed in China. In other situations, the purpose may be acceptable but the means questionable, regardless of whether they are achieved by facial recognition: in the example mentioned above, it may be argued that searching for passengers’ faces in a reservation database and a police database is not an effective means of reducing the risk of a terrorist attack, since a terrorist may have a valid ticket and may not be included in any police database. In addition, this method is ineffective against internal attackers (e.g. railway company employees). The expected benefit of any system based on such a strategy is therefore low. In other cases, it is the use of facial recognition as such, regardless of its actual performance, that can be challenged: for example, the CNIL recently expressed some concerns about using facial recognition for access control in high schools because “the objectives of securing and making it easier to enter these high schools can be achieved by means that are much less intrusive in terms of privacy and individual freedoms, such as a badge” [32]. Woodrow Hartzog’s position [33] also concerns facial recognition as such: according to him, its invisible, ubiquitous and opaque nature, plus the fact that there are already large quantities of photographs available in various databases, is likely to create a generalized sense of surveillance that justifies its absolute prohibition. Finally, certain impacts may be due to the features of a particular system or implementation, such as being too imprecise or biased, or to its context of deployment. The main argument of the CNIL against ALICEM [34] is of this kind: it does not call into question the use of facial recognition but the validity of users’ consent, which is not free if no alternative solution is available. This argument could easily be countered by offering alternative solutions to achieve the same level of safety [35], as suggested by the CNIL itself.
Following the top-down procedure suggested above, each analysis step can be seen as a test to be passed before considering the next level. If, for example, the purpose is deemed unacceptable, it is useless to consider the means to achieve it. Similarly, if the means are not effective, it is useless to consider their realization by facial recognition, and so on.
Comparative approach: another essential dimension of our method is the emphasis on the comparative nature of the analysis: both benefits and risks must be assessed in comparison with alternatives. The initial situation (before any deployment of the system) is generally used as an implicit benchmark, but other options should also be considered at each step: other means to achieve the purpose, other solutions than facial recognition, and other implementations of facial recognition. As an illustration, Figure 2 provides a simplified comparative analysis of our fictitious “train access control” example. At each step, different alternatives are considered and evaluated.
The analysis can also lead to proposals for countermeasures or improvements that could reduce the negative impacts or increase the positive impacts. In other words, the debate must not be closed, reduced to an alternative: to accept or reject the proposed system. Rather, the goal of the approach is to stimulate a critical spirit based on rigorous arguments and to bring out suggestions for alternatives.
Rigorous approach: a final important methodological point concerns the evidence that must be provided to support the arguments or assumptions put forward in the analysis. Too often, discussions about facial recognition are based on positive or negative preconceived ideas, particularly about its supposed effectiveness and the risks it may give rise to. It is therefore important, for each argument put forward in the discussion, to determine its precise status. At least three types of arguments can be distinguished: (1) arguments already supported by experimental measures or results broadly accepted by the scientific community (such as those concerning the risks of bias in algorithms [36]); (2) arguments that are not validated by sufficient studies but that could be tested (such as those concerning the benefits of facial recognition in the police field [37]), which may require experimental deployments in some cases; and (3) arguments that are based on subjective or political positions (such as the argument that the development of facial recognition should not be too constrained so as not to harm innovation, or, conversely, the idea that any deployment, even experimental, should be banned because it would necessarily be used by hidden police) and are not subject to experimental evaluation. In addition, when experiments are conducted to validate hypotheses, they must be carried out independently and follow a precise protocol. We return to this crucial issue in Section 4.
To conclude this overview of the methodology, we would like to emphasize that, apart from the third step, it is not specific to facial recognition and it could also be applied, mutatis mutandis, to any AI or algorithmic system. This generalization will be the subject of future work.
3.2 Detailed presentation
For each of the four levels of analysis, it is necessary to:
- Precisely characterize the system at the considered level (purpose, means, use of facial recognition and implementation).
- Identify all issues (positive and negative impacts, people affected, etc.).
- Analyse these issues (assessment of the severity and likelihood of impacts, their temporary or permanent, reversible or irreversible nature, etc.).
- Consider alternatives or improvements to address the identified issues.
To facilitate the identification and analysis of issues, it may be useful to resort to ethical matrices. Ethical matrices were initially proposed by Ben Mepham in the field of bioethics [38] and they have then been used in other fields such as food or energy. They provide a way to represent concisely all issues for all stakeholders: their lines represent the categories of stakeholders and their columns the potential impacts. An example of ethical matrix is shown in Figure 5 of Appendix 2. Impacts are often grouped into three categories, respectively well-being (health, living conditions, etc.), autonomy (freedom, dignity, etc.) and fairness or justice, but other choices are possible. In particular, the protection of personal data and privacy could be distinguished as a separate issue in the context of the preparation of a Data Protection Impact Assessment (DPIA) under the GDPR [39] or the Law Enforcement Directive [40]. We do not discuss further DPIA in this document because they have already given rise to many publications. In particular, the CNIL has made available several guides and a tool to help data controllers in this task [41]. As discussed in Section 3.1, the general impact assessment described in this document can be used as a preparatory step for a DPIA. Similarly, it can also be useful to conduct the fundamental rights impact assessments called for by the FRA [42].
From an operational point of view, impact assessment should be carried out in a collaborative manner involving all stakeholders, for example by following established participatory procedures (consensus conference, public consultation, etc.). The roles of the stakeholders and technical experts may vary depending on the scope of the project (e.g. specific project or broader debate on facial recognition) and analysis step. For example, citizen participation is essential in steps 2 and 3 (identification and analysis of the issues, at each level) as well as, to some extent, in step 4 (proposals for alternatives or improvements). Technical expertise is essential for the precise characterization of the use of facial recognition and the detailed specification of the system as well as for the suggestion and evaluation of alternatives. The prime contractor must justify the proposed solution, in particular the purpose, the means and use of facial recognition to achieve them.
In the following sections, we provide more details about the impact analysis at the four levels identified above: purpose (section 3.2.1), means (section 3.2.2), use of facial recognition (section 3.2.3) and its implementation (section 3.2.4). We do not discuss the legal aspects in detail here, but they can also be considered at each of these levels, including the compliance with the GDPR, the Law Enforcement Directive, the EU Charter of Fundamental Rights and the European Convention on Human Rights.
3.2.1. Purpose
The purpose is the ultimate objective, the justification for the deployment of the system: therefore, its definition is the basis of the whole analysis. Even if it is more political or economic than technical in nature, it must be defined as precisely as possible in order to allow for the identification and analysis of all issues. For example, if we consider a secure digital identity solution, it is important to know whether its use will be mandatory to get access to certain websites and, if so, what those sites are. The stakes will be very different if the solution is an optional authentication solution to get access to specific government websites such as a tax authority website, or if it is intended to become mandatory for a large number of websites, public or private. In the second case, it would question the notion of anonymity on the Internet. Similarly, the legitimacy of a video surveillance image analysis systems for the purpose of searching may depend on the precise characterization of the notion of “wanted person” (terrorist or criminal on the run, runaway child, missing adult, etc.). Some projects may also look questionable from the outset, politically or legally: this would be the case, for example, of an initiative to prevent certain people from participating in public demonstrations or to identify demonstrators. Moreover, even if a purpose is legitimate, it may be more or less critical and may have different impacts, in the short or long term, for different categories of population.
Examples of key questions to ask at this stage are:
- Is the declared purpose lawful?
- What are the expected benefits of the purpose and what interests does it serve (private or public, state, citizens, etc.)?
- Are the expected benefits of major or relative importance? Is the purpose really a priority?
- What are the possible impacts, positive or negative, in the short and long terms, of the purpose for all stakeholders [43], regardless of the means adopted to achieve it?
- Are the impacts of major or minor importance?
- On the basis of these elements, is the purpose legitimate?
3.2.2. Means to achieve the purpose
The means describes the strategy adopted to achieve the purpose, independently of a particular implementation, by a computer system and/or human operators.
In the train platform example, the means was defined in Section 3.1 as “control, when accessing the platform, that (1) the face of the traveller corresponds to a photograph associated with a valid ticket in the system, and (2) this photograph or the identity of the traveller is not contained in a police database”. The first question to be asked is the effectiveness of the proposed means to achieve the purpose and the availability of any evidence (studies, experiments, etc.) to sustain it. For a better separation of issues, it is assumed at this stage that the means are perfectly implemented. In the example considered here, the proposed means can be challenged because it is ineffective to deal with internal attacks (from a malicious employee or subcontractor) or, in general, threats from persons who are unknown to the police services. Two options are possible at this stage of the analysis: either the means is not subject to revision, it is then considered ineffective and the project should be rejected; or the means can be modified and improved.
In the case of the ALICEM system referred to in Section 3.1, the purpose is to allow users to prove their identity and the means is for users to show something they own (in this case, their passport) and something they are (in this case, his face). This is a case of multi-factor authentication well known in the security field. Depending on the desired level of guarantee, a third factor is sometimes requested (showing that the user knows something, for example a password). This analysis phase could then conclude that the means proposed for ALICEM are appropriate.
After analysing the effectiveness of the proposed means, it is necessary to identify the possible impacts of this means on all stakeholders and on society as a whole (in particular on democracy). In the example of access control to station platforms, we can distinguish, for example, impacts on travellers, railway company employees, the company itself, citizens in general (or society), the State and also technology suppliers. If we consider, for example, travellers, we can identify as positive impact an increased sense of security (which may be justified or not) and as negative impacts, a restriction of the freedom to move anonymously and a feeling of surveillance. With regard to the possible risks, it is important to consider the number of persons included in the police database, the criteria used to include a person, and the type of authorisation, judicial or administrative, necessary to get access to this database. The interested reader can find in Annex 2 a more complete list of possible impacts for different categories of stakeholders.
With regard to possible alternatives to the proposed means, it is possible to imagine, for the previous example, having luggage subject to X-Ray examination and using security gates for passengers (as in airports) without the need to check nominative tickets. It is then necessary to compare the two solutions, considering their positive impacts (particularly in terms of effectiveness) and negative impacts. In this case, it could be argued that this alternative would better achieve the purpose while preserving the freedom to move anonymously. However, it could lead to longer waiting times at boarding. In a real analysis, these arguments should be supported by results or experimental studies which should enable a rigorous comparison of the options. Moreover, the legitimacy of the means and the relevance of the alternatives obviously depend on the purpose itself. Note that the above objection about the train platform access control system would not apply to a project whose purpose, for example, would be to control the access of a restricted area by a few authorised employees. In this case, the database would be limited to a small number of employees.
Examples of key questions to ask at this stage are:
- Does the means effectively accomplish the purpose? What is the evidence to support this?
- What are the possible impacts, positive or negative, in the short and long term, of the means for all stakeholders, regardless of whether facial recognition is used to achieve this means?
- Are these impacts of major or minor importance?
- What could be alternative means of achieving the goal?
- On the basis of these elements, is the means proportionate to achieve the purpose?
3.2.3. Facial recognition to achieve the means
The third level of our analysis framework considers the use of facial recognition technology. The objective at this stage is to describe how facial recognition would be used without going into the details of its implementation, which is analysed at the fourth level. If we continue with the train scenario used above, we can consider that the traveller who arrives at the gate is automatically photographed and his face is compared against the railway company’s reservation database and the police database. The traveller can get access to the train only if he is in the first database and he does not appear in the second one.
Since the way facial recognition is implemented has not yet been introduced, it is assumed at this stage that the implementation is “perfect”, in particular that it is accurate, free of bias and the database is managed in a secure way. The issues addressed at this stage are therefore more theoretical than experimental in nature: they concern the inherent benefits and risks of facial recognition.
As for the previous level, the first question to be asked is whether facial recognition is an effective solution to achieve the proposed means. In the proposed example, the conclusion could be that it is the case. With regard to risks, it is also important to consider the possible drifts or successive extensions to which the processing could give rise. This risk, which is more of a medium or long-term concern, is often mentioned as a key issue by opponents of facial recognition. Such extensions, already mentioned in Section 2.3, may concern both the picture databases, the authorized purposes and the contexts in which the systems are used. Some opponents even argue that this is a deliberate strategy of facial recognition promoters, consisting in using it first in contexts where the purpose seems legitimate and then gradually extend their use. The “slippery slope” argument must therefore be considered seriously, but it must be analysed precisely and in concrete terms to avoid sophisms [44]. With regard to the example of access control to station platforms, it could be argued that there is a significant risk of generalisation to all modes of transport (metro, tram, bus, etc.) that would lead to a total loss of the freedom to move anonymously. Beyond transport, we could also imagine a generalisation to all closed places where people gather (cinemas, theatres, shopping centres, etc.): if these systems are considered effective, why would the protection that they offer be limited to public transports? If this generalisation is not acceptable, where should the red line be set and what safeguards should be provided to ensure that this limit will be enforced? In other cases, it is the databases themselves that could later be extended or cross-referenced with other databases. There is no shortage of examples in recent history to show that this kind of drift is not a fantasy [45]. This “systemic” risk and the consequences of mass surveillance on freedom of expression and democratic life have been widely documented and analysed [46].
It is also necessary to consider the necessity and proportionality of facial recognition. The answer to these questions may depend on many factors, including the scope of application: as mentioned by the ICO, a targeted facial recognition system, limited in time and space, to monitor known suspects is likely to be more justifiable than a large-scale, indiscriminate and permanent deployment [47].
Finally, it is important to consider existing alternatives to facial recognition. In the example of the train platform, one could imagine a solution relying on visual inspections by railway company employees. This control could involve a search for the person’s photograph in the reservation database (based on his or her identity) and a direct comparison with photographs of wanted persons. We could also imagine reading a barcode or QR code that would give access to the photograph associated with the reservation. In any case, the main disadvantage would be a less smooth boarding, and probably a higher cost. The existence of less privacy-invasive alternatives may be a key factor in deciding whether the use of facial recognition is proportionate, as evidenced by the CNIL’s opinion on experiments in high schools in Nice and Marseille, France. In this case, the CNIL held that “the objectives of securing and facilitating access to high schools can be achieved by means that are far less intrusive in terms of privacy and individual freedoms, such as badge control” [48]. In general, as suggested by the EDPS [49], the use of facial recognition for authentication purpose is more proportionate than its use for identification or tracking.
Examples of key questions to ask at this stage are:
- Is facial recognition an effective solution to achieve the means?
- Would its use require the creation or the reuse of a centralized database of images (as opposed to a distributed solution or the use of images remaining on local devices) and what entities would be in control of this database ?
- Would it be used for authentication, identification or tracking ?
- What are the possible impacts, positive or negative, in the short and long term, of facial recognition for all stakeholders? Are they of major or minor importance?
- Is this application of facial recognition likely to lead to extensions or generalizations? What would be their impacts? Can negative effects be prevented or reduced and positive effects promoted or amplified?
- What alternative technologies could be mobilized to achieve the means?
- Based on these elements, is the use of facial recognition proportionate to achieve the means?
3.2.4. Implementation of facial recognition in a particular system
The fourth level of our analysis methodology takes into account the implementation of facial recognition technology. In particular, it analyses the features (and weaknesses or limitations) of the technologies used, the configuration parameters, data, actors, deployment environment, etc. At this stage, it is necessary to evaluate the system as a whole, including the image processing and database components, taking into account the countermeasures put in place, the control and transparency mechanisms, as well as the socio-economic conditions for its deployment.
The benefit of distinguishing risks related to the use of facial recognition from those related to a particular implementation is to separate the fundamental problems raised by the use of this technology from those related to the state of the art at a given time. For example, the analysis may, in some cases, conclude that the use of facial recognition would be acceptable if it could satisfy a set of essential requirements (in terms of performance, reliability, safety, fairness, etc.) but that the existing solutions are not yet mature enough or that the proposed one is not satisfactory.
This phase requires, first of all, to precisely define the system, i.e. its deployment context, the technical specifications of the solution, the configuration parameters, the data used, the actors involved and their roles, etc. It is then necessary to evaluate the “intrinsic” properties of the system, in particular [50]:
- Its performance and reliability, by measuring, for example, false positive rates (when the system “recognizes” a person that should not be recognized) and false negative rates (the system does not “recognize” a person that should be recognized).
- Its security by analysing the confidentiality, integrity and availability properties of the system. In particular, it is necessary to answer questions such as: can the system’s performance be altered by a malicious actor? Have the databases integrity and confidentiality been tested? Is it possible to mislead the facial recognition system? Is it possible to access the configuration system, models or data used? This phase requires assumptions about the potential adversaries (sources of risk), particularly in terms of objectives, capabilities and strategies [51].
- The guarantees on the data used, including the training data and the various databases used by the system, and the protection of users’ privacy.
- The fairness or potential biases of the system, by assessing, for example, error rates for different groups (ethnic, demographic, etc.) of the population.
It is then necessary to evaluate the “extrinsic” properties of the system such as:
- Transparency: are the algorithms, models and data used by the system available (publicly or through restricted access by independent experts)?
- Explainability: are the system’s behaviour and results understandable and explainable to users, especially in the case of false positives or negatives?
Finally, it is necessary to analyse the technical, organizational and legal accountability mechanisms put in place. For example, the following questions should be asked: who are the actors involved and responsible for the system? How can these actors be “accountable” for their actions and to whom? What measures are planned to ensure the oversight of the processing? Do they involve independent third parties, stakeholder representatives, including citizens?
The study of the properties of a system is a very complex and time-consuming exercise. It is important to note that this analysis often depends on multiple parameters such as the algorithm used, the size and quality of the training data (in the case of systems relying on machine learning) or the choice of the confidence threshold. For example, a system could identify a wanted person with a probability of 70% and another one with a probability of 99.9%. If this probability is higher than the confidence threshold, an alert will be issued, otherwise the event will be ignored by the system. As an illustration, Amazon recommends using a 99% confidence threshold for police uses of its Rekognition system but a 80% confidence threshold for less critical applications [52]. Intuitively, a high confidence threshold leads to the detection of very likely events only (low rate of false positives), with the risk of not detecting a significant number of suspects (low true positive rate). The risk of false identification is then limited, at the cost of the efficient of the detection. Conversely, a low confidence threshold makes it possible to identify most of the people wanted, at the cost of a high false positive rate (people wrongly identified). It should also be noted that the performance of a system often depends on the environment in which it is deployed (features of the camera, brightness, angle of view, etc.). A rigorous analysis should therefore include field measurements.
An example of a system whose implementation could be challenged is the French ALICEM application already mentioned above. ALICEM is a mobile phone application developed by the French ministry of internal affairs and the national agency for secure documents (ANTS) in order to allow any individual to prove their identity on the Internet in a secure way. ALICEM uses “real-time” facial recognition to prove that the person who wants to generate a secure identity is the owner of the passport used (by comparing the photograph stored in the passport with those from a video that the user must take and send to ANTS). The security assumption is that only the passport owner can make the video in real time. However, recent results on the production of fake images (“deepfakes“) have shown that it is possible, using open source software, to automatically generate such a video using the face swapping technique [53]. Experiments have shown that recognition systems accept these videos with a probability of more than 84%. An attacker able to use a victim’s passport could then generate a secure digital identity in the victim’s name, which calls into question the security of this application. Another weakness of ALICEM concerns the communication of the video to the ANTS server, whereas the comparison could probably be made locally (on the phone) by the application itself. In addition, it should be noted that facial recognition is only used during the activation phase of the user’s account. Thereafter, the user can simply use the digital identity created during this activation phase, via the ALICEM application, to authenticate himself to the sites he wishes to access. The security of the system therefore depends on the security of the application code and the security of the phone, in particular the Android operating system, which raises doubts about ALICEM’s overall security.
More generally, a facial recognition system is not a technical object that can be analysed in isolation, regardless of the socio-economic context of its deployment. Rather, it is an example of socio-technical system. The analysis of an implementation should therefore consider all its facets, including the sociological (actors concerned, roles and interests in the system, perception of the replacement of human activities by machines, impact on human relations, etc.), economic (development, deployment and maintenance costs, etc.) and strategic (dependence on certain industrial actors or foreign powers, risks of cyber-attacks, etc.) dimensions. These elements must then be evaluated in terms of the declared purpose and assessed in comparison with alternative solutions.
Examples of key questions to ask at this stage are:
- Are the technical specifications of the system known?
- Has the performance (reliability, security, fairness, etc.) of the system been rigorously assessed?
- Has the security of the database(s) been analysed? Are the procedures to manage the database(s) clearly defined, controlled and accountable?
- Is the system transparent and are the results explainable?
- Are accountability measures sufficient?
- What are the possible impacts, positive or negative, in the short and long term, of weaknesses in the implementation of the system for all stakeholders? Are they of major or minor importance?
- Can negative effects be prevented or reduced and positive effects promoted or amplified? Have sufficient countermeasures been put in place?
- What is the financial cost of the system (development, deployment, maintenance, etc.)? Is it acceptable in view of the purpose?
- Could other implementation options offer a better balance between risks and benefits (at an acceptable cost)?
- Based on these elements, is the solution acceptable?
- If not, could other options for implementing facial recognition be acceptable?
4. Conclusion
In this document, we have shown the diversity of facial recognition applications and the variety of parameters to be taken into account when analysing them: type of purpose (security, time saving, convenience, etc.), functionality (identification, authentication, tracking; real-time or offline, temporary or permanent operation, in a geographically limited or larger area, based on a centralised or non-centralised database, etc.), deployment context (consent of the parties involved, role of the stakeholders, judicial authorisation, etc.). We have proposed, in order to analyse the impacts of these systems in a rigorous way, a methodology whose main aspects are summarized in Figure 3.
It should be stressed that, although this document is illustrated mainly with examples of facial recognition applications for public services, similar (or even more) attention should be paid to the uses of facial recognition by private actors. The associated risks can also be very high and the benefits less obvious [54]. Failing to consider commercial applications would lead to the paradoxical situation, such as the one mentioned by Sidney Fussel about the city of San Francisco, where police forces cannot use facial recognition to look for a suspect after a shooting, while a shopkeeper in the city would be authorised to use the same technology to analyse the behaviour of his customers.
It should also be noted that this report does not address the legal aspects of facial recognition: facial recognition systems, because they process personal, and even sensitive, data, are obviously covered by the GDPR and the Law Enforcement Directive. More generally, their fundamental rights implications should be taken into account before their deployment. Even if it is not the main focus of this report, we believe that the methodology suggested here can be profitably used to conduct Data Protection Impact Assessments and fundamental rights impact assessments, which are considered by the FRA as “an essential tool to ensure a fundamental rights compliant application of facial recognition technologies, whatever the context in which it is employed.” [55] For example, some of the arguments used by the FRA concern the purpose (the type of crime targeted by the system, the context – e.g. surveillance of a sport event or a demonstration, etc.), while others are related to the means (e.g. the surveillance of children to prevent serious crime or terrorism), facial recognition itself (e.g. the chilling effect of the use of facial recognition in public places) or its implementation (e.g. risk of being wrongly flagged and the risk of discrimination). As stated by the FRA, “The fundamental rights implications of using facial recognition technology vary considerably depending on the purpose, context and scope of use. Some of the fundamental rights implications stem from the technology’s lack of accuracy […] Moreover, importantly, several fundamental rights concerns would remain even if there were a complete absence of errors”. This comment is in line with our four phase approach. Last but not least, we also believe that the issues at stake go beyond the framework of positive law and privacy protection: as Wojciech Wiewiórowski, the European Data Protection Supervisor, points out, “focusing on privacy issues would be a mistake. This is fundamentally an ethical issue for a democratic society.” [56]
We believe that accountability is key requirement for the deployment of any facial recognition system is [57]. Indeed, it is not uncommon, even in democratic countries, that technologies initially deployed for the purpose of combating terrorism or crime are eventually extended to the surveillance of other categories of persons, such as journalists or activists [58]. Facial recognition is not immune to this risk. Indeed, once images are captured, recorded and potentially analysed, no technical solution can provide an absolute guarantee that the system will not be misused, prove to be less efficient than expected, or even erroneous or, in general, or more generally produce unexpected effects [59]. It is therefore essential to put in place measures requiring any entity exploiting a facial recognition system to report on its use of the system, including the implementation of precise rules for management of databases (procedure for entering a person into the database, cross-referencing with other databases, possibility of contestation, measures to enhance the security of the database, etc.), providing guarantees on the quality of the algorithms (performance, absence of bias, etc.), and recording in a secure manner all uses of the data, the purpose of these uses, the proof of their authorisation, etc. To be effective, such measures should be overseen by a competent independent body capable of providing all stakeholders (including citizens or their organisations) with visibility and guarantees on the use of these systems. Last but not least, the sanctions imposed on entities that do not comply with their obligations should sufficiently deterrent.
This document mainly concerns the development and deployment phases of facial recognition systems. In some cases, field testing may be necessary, in particular to evaluate the performance of the system with regard to the objectives and the possible alternatives. It is important to remember that such field testing must also be subject to an impact assessment [60]. In addition, to be truly useful, they must follow a rigorous protocol and be limited in time. In particular, the objectives of the experiment, the conditions under which it will be conducted, the assumptions of the study, and the criteria to be used for evaluating the results must be clearly defined. Ideally, these experiments should also involve scientists from different disciplines, including computer scientists and sociologists, and be validated by an independent third party. As the CNIL points out, the “legal framework must therefore guarantee the fairness of the trials carried out, the outcome of which should not be prejudged” [61]. In addition, experimentations “should not have the ethical purpose or effect of accustoming people to intrusive surveillance techniques, with the more or less explicit aim of preparing the ground for further deployment.” [62]
In conclusion, we would like to stress that it is necessary to advance the state of the art to improve the confidence that can be placed in facial recognition systems. We believe that several concrete actions are urgent at this stage:
- The definition of a reference framework for conducting impact analyses of facial recognition systems. We hope that this document can contribute to the development of such a reference framework, but it should be defined and issued by an official body. Previous examples of this approach in connected areas include the DPIA methodology and tool proposed by the CNIL [63] and the “algorithmic impact assessment” framework issued by the Canadian government [64].
- The definition of standards or methodologies for the testing, validation and certification of facial recognition systems. These standards should provide guarantees regarding the compliance of these systems with essential requirements, for example in terms of accuracy, absence of bias and database security. In addition, these guarantees should be verifiable by independent third parties. To this end, it is necessary to define standard evaluation schemes in the same spirit as existing information technology security or safety evaluation schemes.
- The definition of a protocol for the experimentation of facial recognition systems in real environments. As mentioned above, laboratory studies are sometimes insufficient and field testing may be necessary to validate certain assumptions. However, there is currently no reference protocol to organize these experimentations.
Experts have an important role to play in these actions but their role should not be limited to the definition of technical standards. As the President of the French Republic, Emmanuel Macron, stressed during his speech at the Global Forum on Artificial Intelligence for Humanity on 30 October 2019, referring to the crucial ethical choices facing our societies today, particularly in the field of artificial intelligence, “this is where the dialogue between political decision-makers, lawyers and scientists is absolutely critical”.
Finally, we would like to conclude that while the current focus on automated facial recognition is important and desirable (in particular because of the unique combination of features presented in Section 2.2), we should not forget that facial recognition is only one technology amongst many others to identify and profile users. As stated by Jake Goldenstein, “The combination of machine learning and sensor surveillance, however, extends that tracking regime with real-time measurement and analysis of how you look, sound, and move, and how your body behaves, through a combination of biometrics (measurement of physical and behavioral characteristics), anthropometrics (measurement of body morphology), and physiometrics (measurement of bodily functions like heart rate, blood pressure, and other physical states). In the world of machine learning and statistical pattern analysis, you don’t need a face to identify a person”[65]. Focusing on facial recognition shold not distract us from other tracking and profiling technologies and from the structural question of whether governments or companies should be building massive databases of personal information in the first place. It is important to bear the broader picture in mind.
Acknowledgements
The authors would like to thank their reviewers, in particular Clément Henin and Vincent Roca, for their constructive comments on an earlier version of this document
Appendix 1: Examples of applications of facial recognition
Facial recognition is a generic term that includes facial recognition and facial authentication [66].
- Facial identification consists in establishing the identity of a user, i.e. finding this identity from one or more photographs of his face. During identification, a photograph of the user is taken [67]. A template of his face is extracted from it and used to search, in a list containing N entries, for the identity that corresponds to this face. It is therefore a (1:N) operation in the sense that a person’s photograph is compared with N other photographs. It is assumed here that the link between faces and identities was made beforehand to constitute the list.
- Facial authentication (1:1) allows the user to provide a proof of his identity. During facial authentication, a photograph of the user is taken, a template of his face is extracted from it and it is used to verify, by comparison, that it corresponds to the one associated with his identity. It is therefore a (1:1) operation in the sense that the photograph of a person is compared with another photograph (supposed to be of the same person).
The security of facial identification or authentication is more or less strong depending on the performance of the image comparison algorithm and the quality of the photographs. It also depends strongly on the guarantee that the face of the photograph taken really belongs to the person who wants to be identified or authenticated. In the case of the front door of a school or a photograph taken by a police officer, this guarantee is relatively high. In the case of a selfie taken by the user with his phone, this guarantee is low because the user can use someone else’s photograph. Additional mechanisms, such as liveness tests, must be used.
Applications of facial recognition can be categorized by considering (1) the entries that are provided by the user at the time of processing (e. g. via a badge or passport), (2) the entries captured by the system (typically a video or photographs), (3) the data stored in a centralized database, (4) the operating mode (real-time or retrospective) and (5) the system outputs. Figure 4 shows some examples of applications classified according to these criteria:
- A secure online authentication system, such as ALICEM, allows a user to generate a secure digital identity remotely [68]. Identification is carried out by presenting the passport. The system extracts the information from the passport (identity and a photograph of the holder) and asks the user to take a video of his face. The information is sent to a server that compares the person’s face on the video and the passport photograph. If successful, the user is authenticated. This system does not require a centralized image database.
- The train access control system, described in Section 3.1, is a system that identifies and authenticates a passenger for authorization purposes. Facial recognition is used to identify the person. The system takes one or more photographs of the face of the person entering the access portal and searches for the face in the database of registered travellers. When the person has been identified, the system checks that he or she is authorized to take this train (i.e. has a ticket and does not appear in the list of wanted persons). The system operates in real time.
- An access control system can also combine the use of a badge, or more generally a “token” [69], and facial recognition. In this scenario, the badge is used to identify the user and facial recognition is used to authenticate the user. It is therefore a facial authentication system. More precisely, the photographs captured by the system are compared with those associated with the user’s badge reference in a centralized database.
- An identification system, such as the one that could be used by the police when they arrest a person who does not want to reveal their identity, uses facial recognition for identification purposes. The system takes photographs of the person to be identified and compares them with those in its internal database (e.g. database of wanted persons). This identification can take place in real time or a posteriori (on images that have been recorded). If an entry is found, the identity associated with that face in the database is returned.
- A system for searching for people (lost children, criminals,) in a public space captures images in real time and checks if the faces that appear on these images correspond to wanted people. Technically, this consists of comparing the faces captured with those in a centralized database (the database of wanted persons). Facial recognition is used for identification purpose.
- A tracking or tracing system uses several target faces (e. g. the faces of suspects to be tracked) and searches for these faces in other recorded images, e. g. from a video surveillance system, or captured in real time. Facial recognition is used here for comparison purpose; the objective is not to identify a person, but to find them in several photographs.
APPENDIX 2: Ethical matrices
The goal of each of the four phases described in Section 3.1 of this document is to identify the potential impacts, both positive and negative, of a facial recognition system. Ethical matrices can be a useful tool to guide these analyses and to provide a synthetic view of their results. The lines of an ethical matrix represent the stakeholders and their columns represent the issues or types of impacts to be considered. Impacts are often grouped into three categories: well-being (health, living conditions, etc.), autonomy (freedom, dignity, etc.) and fairness or justice. However, other choices are possible depending on the context and the values or ethical families to which stakeholders wish to refer. It should be noted that this type of matrix can also be used to conduct a Data Protection Impact Assessment or a fundamental rights impact assessment, as called for by the FRA. By default, privacy impacts can be categorized in one of the three columns (well-being, autonomy and fairness) but it is also possible to consider a dedicated column. Similarly, the fundamental rights most affected by facial recognition technologies, as identified by FRA, can fit in such matrices. For example, privacy, non-discrimination and freedom of expression appear in the example below; the protection of the rights of the child and elderly people is not mentioned here but it could also be highlighted through a dedicated line in the matrix.
Figure 5 represents a possible ethical matrix resulting from the analysis of the case study referred to in this document, considering the purpose of “preventing a terrorist from committing an attack on a train” and as means, the “control, when accessing the platform, that (1) the face of the traveller corresponds to a photograph associated with a valid ticket in the system, and (2) this photograph or the identity of the traveller is not contained in a police database”. Figure 5 is of course not intended to be exhaustive, the purpose here being only to illustrate the use of ethical matrices. In each column, the items marked “+” represent positive impacts and the items marked “-” represent negative impacts.
It should be noted that ethical matrices do not associate levels of likelihood or severity with the identified impacts. For example, in the well-being column, there can be a positive impact on passengers if the boarding procedure is faster and a negative impact in the event of malfunction; similarly, increased controls can produce a sense of security for some people and a sense of insecurity for others. It is in the final phase, the deliberation, that the likelihood, severity and priorities between impacts must be discussed, in a process that should involve all stakeholders.
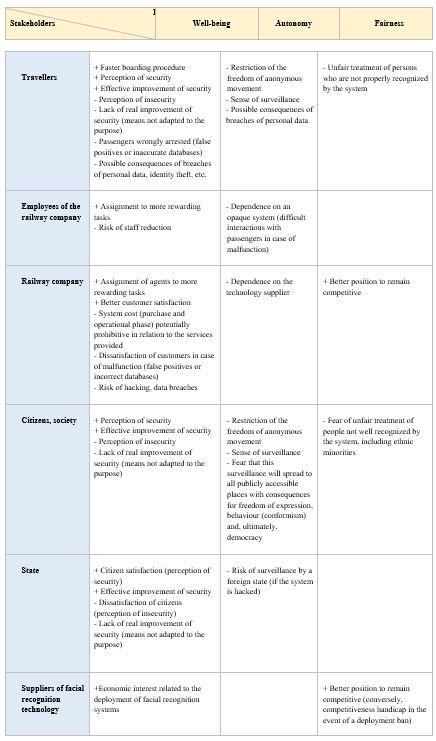
End Notes
[1] [In French] Comment des villes « hyper connectées » contrôlent l’espace public, Le Monde, 19 December 2018, https://www.lemonde.fr/economie/article/2018/12/19/au-nom-de-la-smart-city-des-villes-sous-surveillance_539952 7_3234.html.
[2] How facial recognition makes you safer, James O’Neill, New York Times, 9 June 2019. https://www.nytimes.com/2019/06/09/opinion/facial-recognition-police-new-york-city.html.
[3] https://www.cnil.fr/fr/la-cnil-appelle-la-tenue-dun-debat-democratique-sur-les-nouveaux-usages-des-cameras-video.
[4] First report of the Axon AI & Policing Technology Ethics Board, June 2019.
[5] Facial recognition is the perfect tool for oppression, W. Hartzog, E. Selligner, Medium.com, http://cyberlaw.stanford.edu/publications/facial-recognition-perfect-tool-oppression.
[6] https://www.cnil.fr/en/facial-recognition-debate-living-challenges
[7] https://www.technologyreview.com/f/614469/france-plans-to-use-facial-recognition-to-let-citizens-access-government-services/
[8] For example, it is important to distinguish the perception of risks from the reality of these risks. See, for example: https://papers.ssrn.com/sol3/papers.cfm?abstract_id=2508019.
[9] https://www.cnil.fr/sites/default/files/atoms/files/facial-recognition.pdf
[10] Although the examples considered in this note are based on some recent developments, they do not necessarily correspond to real systems (for which little technical details are generally available). These examples should therefore be considered as hypothetical systems provided for illustrative purpose.
[11] That is, whose faces are compared.
[12] This use is sometimes referred to as “facial surveillance”: Here’s a way forward on Facial Recognition, October. 31 2019, https://www.nytimes.com/2019/10/31/opinion/facial-recognition-regulation.html.
[13] This use is sometimes referred to as “facial identification”.
[14] This distinction emerged in the United States in particular after several cities decided to ban the use of all forms of facial recognition by municipal services, including the police: some called for a distinction to be made between these two forms of use and for the possible use of the second mode of operation in order not to deprive the police of effective tools.
[15] https://edps.europa.eu/node/5551.
[16] https://eur-lex.europa.eu/legal-content/EN/TXT/HTML/?uri=OJ:L:2016:119:FULL&from=DE.
[17] See for example : https://www.nytimes.com/2020/01/18/technology/clearview-privacy-facial-recognition.html, https://www.nytimes.com/interactive/2019/10/11/technology/flickr-facial-recognition.html, https://www.vice.com/en_us/article/a3x4mp/microsoft-deleted-a-facial-recognition-database-but-its-not-dead, or https://megapixels.cc/datasets/megaface/.
[18] The perpetual line-up. Unregulated police face recognition in America, Georgetown Law Center on Privacy & Technology, octobre 2016. https://www.perpetuallineup.org/sites/default/files/2016-12/The%20Perpetual%20Line-Up%20-%20Center%20on%20Privacy%20and%20Technology%20at%20Georgetown%20Law%20-%20121616.pdf.
[19] Traitements Électroniques Sécurisés: Secure Electronic Identity Documents.
[20] Solutions exist for some applications andservices: https://www.theregister.co.uk/2019/06/10/microsoft_windows_photos_facial_recognition_consent/. [21] Gender shades: intersectional accuracy disparities in commercial gender classification, J. Buolamwini, G. Gebru, Machine Learning Research, No 81, 2018, http://proceedings.mlr.press/v81/buolamwini18a/buolamwini18a.pdf. AI Now Report 2018, https://ainowinstitute.org/AI_Now_2018_Report.pdf. AI experts question Amazon’s facial-recognition technology, New York Times, 3 April 2019, https://www.nytimes.com/2019/04/03/technology/amazon-facial-recognition-technology.html. Amazon’s face recognition falsely matched 28 members of congress with mugshots, ACLU, https://www.aclu.org/blog/privacy-technology/surveillance-technologies/amazons-face-recognition-falsely-matched-28.
[22] Facial recognition is the perfect tool for oppression, W. Hartzog, E.Selligner, Medium.com, http://cyberlaw.stanford.edu/publications/facial-recognition-perfect-tool-oppression.
[23] https://www.theguardian.com/technology/2019/jun/07/facial-recognition-technology-liberty-says-england-wales-police-use-should-be-banned.
[24] Many cases have been revealed. See for example: https://www.nytimes.com/2020/01/18/technology/clearview-privacy-facial-recognition.html, https://www.nytimes.com/interactive/2019/10/11/technology/flickr-facial-recognition.html, https://www.vice.com/en_us/article/a3x4mp/microsoft-deleted-a-facial-recognition-database-but-its-not-dead, or https://megapixels.cc/datasets/megaface/. In France, an article of the finance bill was also recently adopted by the National Assembly, despite the reservations of the CNIL: its purpose is to enable tax and customs services to collect data on social networks to detect certain types of frauds: https://www.theguardian.com/world/2019/oct/01/french-plan-to-scan-social-media-for-tax-causes-alarm
[25] For example as it happened in France with the National DNA file (the “FNAEG” file) which has been extended by several laws or with the Secure Electronic Identities (the “TES” file) and the Criminal Records Processing files (the “TAJ” file): https://www.cnil.fr/fr/donnees-genetiques-les-reserves-de-la-cnil-sur-lamendement-portant-sur-lelargissement-du-fnaeg and https://www.laquadrature.net/2019/11/18/la-reconnaissance-faciale-des-manifestants-est-deja-autorisee/.
[26] https://www.aclu.org/blog/privacy-technology/surveillance-technologies/how-tsas-facial-recognition-plan-will-go-far.
[27] For example, to coordinate crews in the field or to monitor major events in real time in the case of the Nice urban supervision centre: https://www.nice.fr/fr/securite/le-centre-de-supervision-urbain.
[28] Face Off, https://www.eff.org/wp/law-enforcement-use-face-recognition.
[29] http://wearcam.org/acmmm2004sousveillance/mann.pdf.
[30] How facial recognition could tear us apart, Judith Donah, interview in Medium, https://onezero.medium.com/how-facial-recognition-tech-could-tear-us-apart-c4486c1ee9c4.
[31] See the ethical matrix in Appendix 2.
[32] [In French] Lycées : la CNIL précise sa position, 29 October 2019, https://www.cnil.fr/fr/experimentation-de-la-reconnaissance-faciale-dans-deux-lycees-la-cnil-precise-sa-position.
[33] Facial recognition is the perfect tool for oppression, W. Hartzog, E. Selligner, Medium.com, http://cyberlaw.stanford.edu/publications/facial-recognition-perfect-tool-oppression.
[34] ALICEM is a secure identity management system (to be deployed in France) relying on
facial recognition to create digital identities.
[35] For the creation of a digital identity with a high level of guarantee (in the sense of the e-IDAS Regulation).
[36] J. Angwin, J. Larson, S. Mattu, L. Kirchner, “Machine bias: There’s software used across the country to predict future criminals. And it’s biased against blacks”, ProPublica, 23 May 2016; https://www.propublica.org/article/machine-bias-risk-assessments-in-criminal-sentencing. W. Flores, K. Bechtel, C. T. Lowenkamp, “False positives, false negatives, and false analyses: A rejoinder to Machine bias: There’s software used across the country to predict future criminals. And it’s biased against blacks”, Fed. Prob. 80, 38 (2016).
[37] Face off. The lawless growth of facial recognition in UK policing, Big Brother Watch, https://bigbrotherwatch.org.uk/wp-content/uploads/2018/05/Face-Off-final-digital-1.pdf.
How facial recognition make you safer, James O’Neill, New York Times, 9 June 2019, https://www.nytimes.com/2019/06/09/opinion/facial-recognition-police-new-york-city.html.
[38] See for example: The ethical matrix – A tool for ethical assessments for biotechnology, E.-M. Forsberg, Golbal Bioethics, Vol. 17, 2004 ; ou Ethical matrix, Food Ethics Council, https://www.foodethicscouncil.org/uploads/publications/Ethical_Matrix_1.pdf.
[39] https://eur-lex.europa.eu/legal-content/EN/TXT/HTML/?uri=CELEX:02016R0679-20160504&from=EN
[40] https://eur-lex.europa.eu/legal-content/EN/TXT/HTML/?uri=CELEX:32016L0680&from=EN
[41] https://www.cnil.fr/en/privacy-impact-assessment-pia
[42] Facial recognition technology : fundamental rights considerations in the context of law enforcement, FRA Focus, November 2019 : https://fra.europa.eu/en/publication/2019/facial-recognition
[43] Stakeholders are defined as all entities, persons or groups of persons, who may be affected by a system, directly or indirectly, in an active (sponsor, developer, operator, user, etc.) or passive (citizen, passenger, etc.) manner. The state or society can also be included as a stakeholder (see Annex 2).
[44] Stakeholders are defined as all entities, persons or groups of persons, who may be affected by a system, directly or indirectly, in an active (sponsor, developer, operator, user, etc.) or passive (citizen, passenger, etc.) manner. The state or society can also be included as a stakeholder (see Annex 2).
[45] The French national DNA database (FNAEG), already mentioned, provides a prime example: created in 1998 to centralize the fingerprints of persons convicted of extremely serious offences (murder of a minor person preceded or accompanied by rape, torture or barbaric acts, etc.), it has been successively extended to include nearly three million DNA profiles in 2018. See also note 24 for the TES and TAJ files.
[46] Chilling Effects: Online Surveillance and Wikipedia Use, Jon Penney, Berkeley Technology Law Journal, vol. 31, No1, https://papers.ssrn.com/sol3/papers.cfm?abstract_id=2769645##. Under surveillance: examining Facebook’s spiral of silence effects in the wake of NSA Internet Monitoring, Journalism & Mass Communication Quartely, Vol. 93(2), 2016. https://journals.sagepub.com/doi/abs/10.1177/1077699016630255.
[47] https://ico.org.uk/media/about-the-ico/documents/2616184/live-frt-law-enforcement-opinion-20191031.pdf
[48] [In French] Lycées : la CNIL précise sa position, 29 octobre 2019, https://www.cnil.fr/fr/experimentation-de-la-reconnaissance-faciale-dans-deux-lycees-la-cnil-precise-sa-position.
[49] https://edps.europa.eu/node/5551.
[50] For more information, see https://www.europarl.europa.eu/stoa/en/document/EPRS_STU(2019)624261.
[51] Differences of opinion often arise from differing assumptions, implicit or explicit, about sources of risk. For example, opponents of deployments of CCTV or facial recognition systems often assume that the operator, e.g. the city, is not trustworthy and may be tempted to use the system for other purposes, or even modify it to allow diversions. The question of the adversary model to be considered in a risk analysis is therefore essential and must be discussed.
[52] https://aws.amazon.com/blogs/aws/thoughts-on-machine-learning-accuracy/.
[53] https://arxiv.org/abs/1910.01933.
[54] See for example : https://www.nytimes.com/2019/11/08/realestate/are-my-neighbors-spying-on-me.html.
[55] Facial recognition technology : fundamental rights considerations in the context of law enforcement, FRA Focus, November 2019 : https://fra.europa.eu/en/publication/2019/facial-recognition
[56] https://edps.europa.eu/node/5551.
[57] It should be noted that we use the term “accountability” here in a general sense, which is less restrictive than the definition of the GDPR. The GDPR defines the term as the fact that the controller is responsible for compliance with the principles relating to the processing of personal data as defined in Article 5 thereof. See in particular: Strong Accountability: Beyond Vague Promises. Denis Butin, Marcos Chicote, Daniel Le Métayer, in Reloading Data Protection: Multidisciplinary Insights and Contemporary Challenges, Springer, 2014. https://hal.inria.fr/hal-00917350/file/cpdp2013-bcm-strong_accountability_v4.pdf.
[58] https://www.nytimes.com/2019/11/09/technology/nso-group-spyware-india.html.
[59] Many examples of abuse have been revealed, such as the FBI case described by the ACLU: https://www.aclu.org/news/privacy-technology/the-fbi-is-tracking-our-faces-in-secret-were-suing/.
[60] This impact analysis should take into account the specific conditions of the experimentation, in particular the possibility to obtain the effective consent of the persons involved, the number of persons concerned, the duration of the experimentation and the importance of the expected results.
[61] https://www.cnil.fr/sites/default/files/atoms/files/facial-recognition.pdf
[62] In this respect, we can quote as a perfect counter-example the test carried out during the Nice carnival in February 2019: https://www.documentcloud.org/documents/6350838-Bilan-Reconnaissance-Faciale.html.
[63] https://www.cnil.fr/en/privacy-impact-assessment-pia
[64] https://www.tbs-sct.gc.ca/pol/doc-fra.aspx?id=32592
[65] https://www.publicbooks.org/facial-recognition-is-only-the-beginning/
Bruce Schneier argues along the same lines in a recent paper in The New York
Times: https://www.nytimes.com/2020/01/20/opinion/facial-recognition-ban-privacy.html
[66] In addition, as discussed later, facial recognition can also be used to track a person, without necessarily identifying them. The face is then used as an “index”.
[67] In practice, several pictures can actually be captured, for example via a video
camera.
[68] Therefore, this is not facial identification.
[69] Object owned by the person, which can take various forms: badge, card, mobile phone, etc.
Cite the article : Claude Castelluccia, Daniel Le Métayer, Inria. Impact Analysis of Facial Recognition: Towards a Rigorous Methodology. 2020.hal-02480647 https://hal.inria.fr/hal-02480647/document
Download the PDF on hal : https://hal.inria.fr/hal-02480647/document
These statements are attributable only to the author, and their publication here does not necessarily reflect the view of the other members of the AI-Regulation Chair or any partner organizations.